Safran Aircraft Engines - Applying a Big Data Strategy to Improve Planning and Enhance Employee and
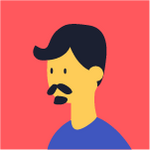
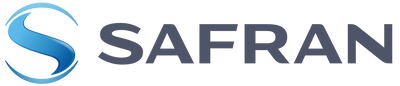
Name:
David Pauline
Karen Jalabert
Myriam Soulimani
Hugo Wiesenfeld
Mael Guyot
Country: France
Organization: Safran Aircraft Engines
The Sales Administration (MSA) department has more than 54 customers in more than 18 countries for 1.3 billion euros in turnover and nearly 60 employees. The MSA department wonders about the performance of its deliveries, 20% of which are delivered out of time, and the management of returns, which costs 1.5 million euros every year. The MSA must guarantee the delivery of products and services in order to make the ROFO (ROlling FOrecast) more reliable. Delivery reliability is indeed a major issue for SAE, which is thinking of improving its business model by exploiting a considerable amount of data. The MSA department must guarantee the delivery of products and services on time, in order to make the ROFO more reliable.
Awards Categories:
- Responsible AI
- Most Impactful Transformation Story
- Most Extraordinary AI Maker(s)
Business Challenge:
Our "Guarantee delivery on time and make the ROFO more reliable" project is designed for the Sales Administration Department, which can no longer tolerate blockages and malfunctions that impact delivery times and therefore customer satisfaction and the failure of our turnover.
This innovative improvement project will allow us to:
- Improve delivery planning.
- Make our quarterly turnover forecasts.
- Become more reliable to satisfy our customers by respecting our commitments.
- Improve the image of the Sales Administration Department.
Faced with these challenges, the Sales Administration Department has chosen to apply a Big Data strategy (predictive analysis) as well as Lean & Agile approaches in order to improve its planning and enhance the employee and customer experiences, by monitoring process data and especially their level of quality.
Business Solution:
Innovation
Using AI technology developed with Dataiku, the project team has developed algorithms that allow it to predict with an accuracy of more than 90% the blockages made in the coming days as well as the delivery date when ordering. AI is used to analyze more than 200 variables, including the address, the contents of the packages, and the supply times to make predictions.
Result
This predictive analysis process, applied to the M53 France (Mirage 2000 engine) and the M88 Qatar (Rafale engine), made it possible to reduce blockages by 50% and gain four points in terms of OTD (On Time Delivery). It has since been generalized to other engine programs and geographical areas.
Results (can be generalized in all of Safran)
Maintenance issues often block the delivery process and create defects in terms of time and quality. By collecting data from different data sources (e.g., ERP), we can predict problems identified in the past and therefore act upstream, even before the problem occurs. This predictive maintenance makes the process flow and helps us avoid quality and delay problems.
Business area enhanced: Manufacturing/Supply Chain/Supplier Management/Service Delivery
Use case stage: Built & Functional
Value Generated:
This project has allowed us to save a significant amount of money in terms of ROI. Furthermore, it’s helped us to meet all of the stakes outlined earlier: our delivery planning has been improved, our quarterly turnover forecasts are more reliable, we’re satisfying our customers by respecting our commitments, and we’ve homogenized the ADV profession and improved its image.
Focusing on the improved reliability of our delivery forecasts, it’s also enabled us to meet two key objectives. Knowing about issues helps us to alert the supply chain and notify our customers, and as a result, we’ve reduced stoppages in the process by 50%. What’s more, knowing about the delivery date helps to make the ROFO more reliable and to warn our partners and customers, allowing us to go from an 80% to an 85% compliance rate.
Finally, to achieve the objective of improving the quality of the measurement system, we’ve implemented data governance. This rests on four pillars:
- Improve data knowledge Inventory data
- Present common and shared definitions.
- Build the data dictionary.
- Define data lifecycle management.
- Set up a mapping of data and their uses. - Strengthen data quality
- Define the level of quality desired for each piece of data and the actions to be implemented.
- Set up rules and procedures, as well as regular audits.
- Write a data quality monitoring plan.
- Develop a heat map to identify areas at risk and allocate resources. - Improve data sharing and pooling of data projects
- Put in place a framework to promote the use of data and share it.
- Set up a portfolio of use cases in order to capitalize on experiences and create value.
- Put in place a framework to promote the use of data and share it. Set up a portfolio of use cases in order to capitalize on experiences and create value. - Democratize data
- Create the conditions allowing employees to understand the scope of data, empower them, and encourage them to improve existing processes, or create new ones.
- Raising awareness (use cases, benefits, tools, etc.)
- Training (data scientist, data engineer, immersion in the data lab, tools, community, network, etc.)
- Communication (events, Station D, games, etc.)
Value Brought by Dataiku:
Thanks to Dataiku, we not only improved our data knowledge but also helped our colleagues to better understand the process and generally upgrade their technical skills. It’s helping to pave the way for data democratization across the organization, with many colleagues able to use Dataiku without formal training, and everyone able to understand what they’re doing despite coming from varying technical backgrounds.
This process has inspired many people within the organization to become more data-driven in their day-to-day work. Coming with different technical skill sets, many are moving from data observers to innovators, while others are going from simply experimenting with data to becoming data pioneers. While many still consider themselves beginners at data science, throughout this process, they’ve already had a number of achievements with Dataiku.
Value type:
- Improve customer/employee satisfaction
- Increase revenue
- Reduce cost
- Reduce risk
- Save time
- Increase trust
Value range: Hundreds of thousands of $