Custom Code Metric
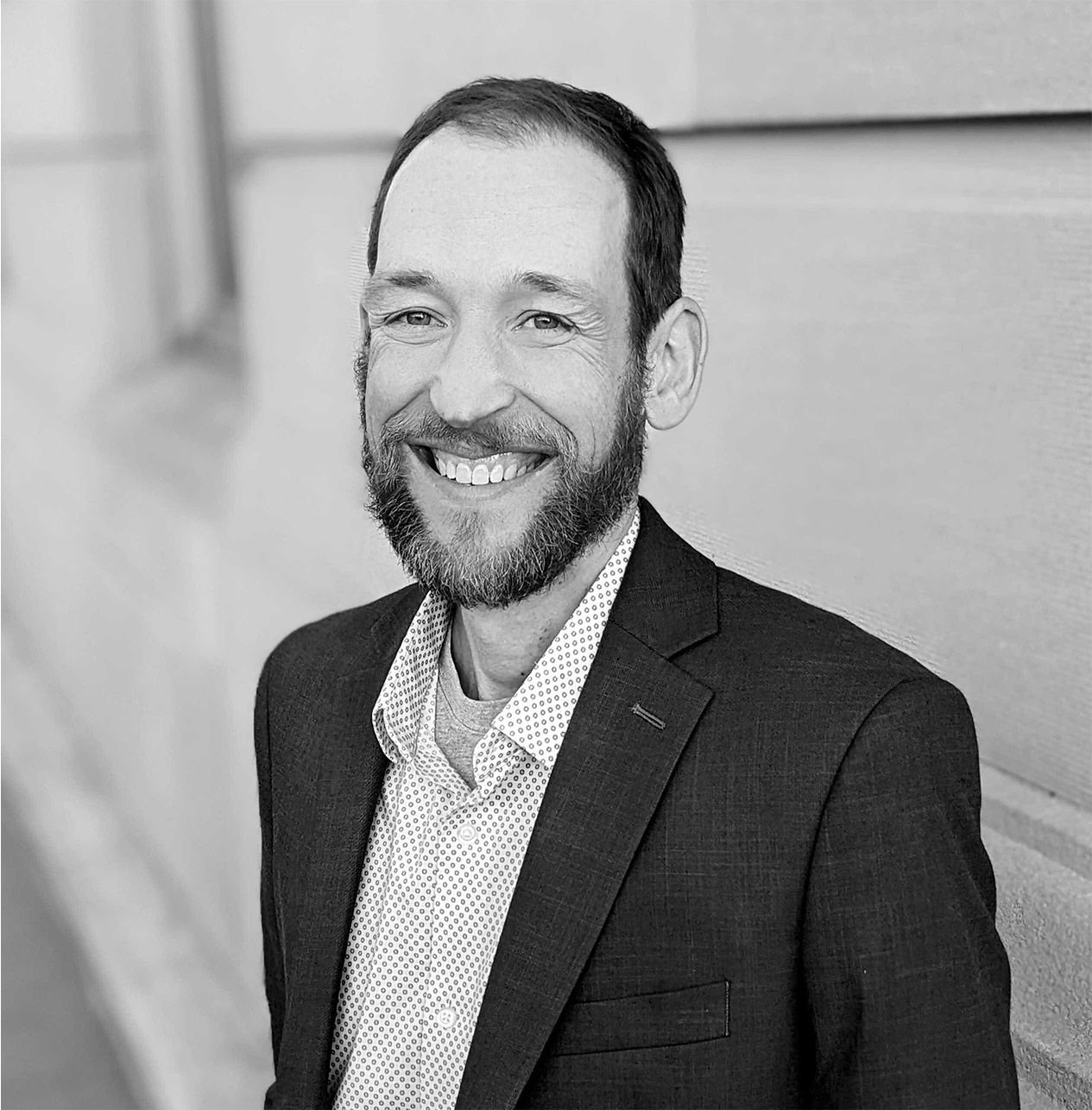
I'm attempting to make the best use of the "Custom Code" option for hyperparameter optimization and have a few questions. For reference, here are the comments on how to write the custom function:
# - y_pred is a numpy ndarray with shape: # - (nb_records,) for regression problems and classification problems # where 'needs probas' (see below) is false # (for classification, the values are the numeric class indexes) # - (nb_records, nb_classes) for classification problems where 'needs probas' is true
- No real issues when "needs_probas" is false. By appending y_pred as a column to X_valid, I'm able to see which rows were predicted as "True" for my binary classification problem (for a given threshold)
With "needs_probas" set to true, I run into some problems.
- It appears that the shape of y_pred is different depending on whether the model is training or scoring. Here's the code I've implemented that seems to solve the problem (again for a binary classification problem). I'm wondering if this should be necessary or if I'm missing something?
if len(np.shape(y_pred)) == 2: # scoring the model ds['probas'] = y_pred[:,1] else: # training the model ds['probas'] = y_pred[0:]
- With "needs_probas" false, the scoring seems to be dependent on the threshold (a row's prediction will be "true" when the proba is above a threshold) and with "needs_probas" false, it appears that the threshold is not provided to the scoring function. Is this correct and expected or am I missing something? Maybe the "needs_threshold" property just isn't implemented in DSS for binary classifications? (https://scikit-learn.org/stable/modules/generated/sklearn.metrics.make_scorer.html)
Best Answer
-
Hi,
you stumbled indeed on a not-so-nice behavior of the custom scoring handling, which passes the full output of predict_probas when doing the final scoring, and only the second column (the positive case) when doing hyperparameter search and k-fold. Your solution is essentially the best one can come up with.
You needn't worry about the threshold, as it is computed after scoring with your code: DSS will try different threshold values and call your scoring code with a single-column corresponding to the positive case.
Answers
-
Hi @rmoore
,This has been fixed in release 8.0.2 :
More precisely, the custom metric function can now correctly assume a
y_pred
shape of(N, 2)
in the case of binary classification withneeds_proba == True
, when performing a hyperparameters searchCheers
-
Hello, I am trying to create customer metrics to return precision score for first 100 predictions. Code is below:
File "<string>", line 23, in score IndexError: too many indices for array
I am getting following error:
import pandas as pd from sklearn.metrics import precision_score def score(y_valid, y_pred): """ Custom scoring function. Must return a float quantifying the estimator prediction quality. - y_valid is a pandas Series - y_pred is a numpy ndarray with shape: - (nb_records,) for regression problems and classification problems where 'needs probas' (see below) is false (for classification, the values are the numeric class indexes) - (nb_records, nb_classes) for classification problems where 'needs probas' is true - [optional] X_valid is a dataframe with shape (nb_records, nb_input_features) - [optional] sample_weight is a numpy ndarray with shape (nb_records,) NB: this option requires a variable set as "Sample weights" """ scoring = pd.DataFrame() scoring['actual'] = y_valid scoring['probability'] = y_pred[:, 1] scoring = scoring.sort_values(by = 'probability', ascending = False) top_100 = scoring.iloc[:100] pr_score = precision_score(top_100['actual'], top_100['probability']) return pr_score
-
Hello, where you pass needs_proba parameter?
-
Hi, from contacting Dataiku I have found that this feature will be available within Dataiku 11.
-
Hello, https://doc.dataiku.com/dss/latest/release_notes/index.html, it seems that DataIku 11 is already released. Correct me if I am mistaken