SLB HR - Talent Acquisition Enablement with Machine Learning
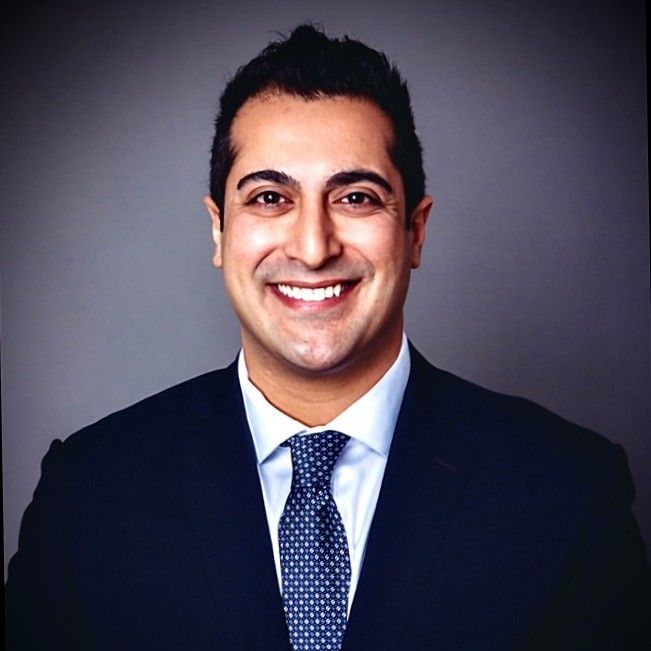
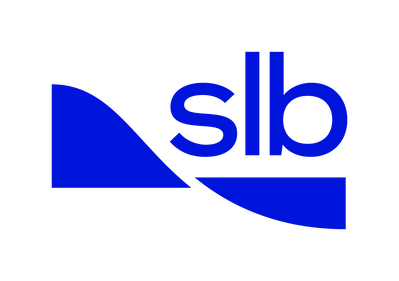
Team members:
Modhar Khan - Head of People Analytics
Richard De Moucheron – Director Total Talent Management
Wesley Noah – Global Compliance Managing Counsel Operations
Sejal Sagar Mehta – Application Engineer
Sudeep Goswami – HR Applications Manager
Ryan Stewart – Global Talent Acquisition Planning Manager
Sonia Badilla - Talent Acquisition Manager Western Hem
Philip Irele Evbomoen - Talent Acquisition Manager - Eastern Hem
Beth Kremer – North America Recruiting Manager
Zhi Chi – Data Engineer HRIT
Simon Spero (Dataiku) - Senior Enterprise Customer Success Manager
Country:
United States
Organization:
SLB
Description:
SLB is a technology company that partners with customers to access energy. Our people, representing over 160 nationalities, are providing leading digital solutions and deploying innovative technologies to enable performance and sustainability for the global energy industry. With expertise in more than 120 countries, we collaborate to create technology that unlocks access to energy for the benefit of all.
Awards Categories:
- Responsible AI
- Value at Scale
Challenge:
Every year, more than 500k candidates apply to SLB across the globe. With our PeopleFirst Strategy, we made a commitment towards improving Diversity & Inclusion in everything we do as a company.
Our Talent Acquisition team had stretched investment and resources to vet these candidates, match them to business demand, and do all of that efficiently with the utmost compliance.
The challenge in using AI & ML was to ensure that it will not have any negative impact on the candidates and to continuously monitor such models that can be vetted and improved, in case they generate any bias against any class. After vetting many ready-made solutions, we found that they do not cover the complexities in 80+ countries, nor the number of profiles we hire for.
Solution:
- Complex data engineering:
Making the data ready for exploration was a complex process as it involved many internal and external data sources, as well as numerous engineering steps and feature generations. With Dataiku, we were able to do that at scale quickly and in a quality manner.
See example of a project showing Dataiku’s ability to handle complexity at scale:
- Ensemble modeling:
From advanced embedding models for text and features extraction to probabilistic predictive workflow, Dataiku was able to handle customizations needed in our ML workflows seamlessly.
- Model deployment:
The API deployer proved to be an efficient and cost effective feature, without requiring to add additional infrastructure in the pipeline.
- Collaboration and adoption:
Recruiters were able to interact with the predictions and provide feedback in a true collaborative manner.
Impact:
Pilot results (Q2-Q3 2021):
- The ensemble model developed was able to rank 10,000 applicants with 82% agreement on the output by a committee of recruiters and managers.
- The API deployed and connected to the candidate processing system is now in the test phase, and we look to deploy it in select countries by mid-year.
- We estimate that this will reduce the time of processing candidates by more than 80%, while providing better experience to applicants with timely feedback.
- This will also support agility in responding to critical business needs.