ZS Associates - Personalized Client Engagement Transformation Experience at Scale for Highest Impact
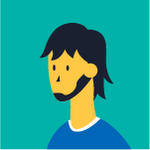
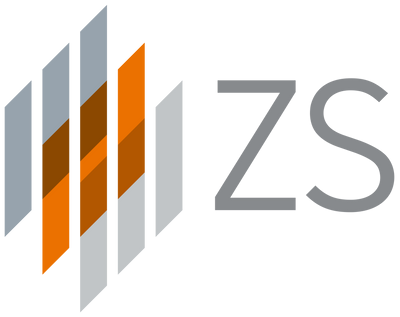
Team members:
- Vikesh Doshi
- Sukesh Sogani
- Vishal Shrivastava
- Subbiah Sethuraman
- Aditya Yadav
- Kratika Saxena
- Parikshit Goutam
- Nihal Mehta
- Ashish Satya Verma
- Utkarsh Jain
- Shubham S Tiwari
- Pratip Khandelwal
Country: India
Organization: ZS Associates
ZS is a management consulting and technology firm focused on transforming global healthcare and beyond. We leverage our leading-edge analytics, plus the power of data, science, and products, to help our clients make more intelligent decisions, deliver innovative solutions and improve outcomes for all. Founded in 1983, ZS has more than 12,000 employees in 35 offices worldwide. To learn more, visit https://www.zs.com/.
Awards Categories:
- Best MLOps Use Case
- Best Data Democratization Program
- Best ROI Story
Business Challenge:
Traditional customer engagement approaches, through various channels, have been product-centered and disjointed, leading to non-personal experiences. This reduces the impact of commercial actions and overall impressions, resulting in fewer patients accessing necessary therapies. To address this, adopting an Omnichannel approach with a customer-centric focus is essential.
ZS has successfully launched the “Client Engagement Transformation” for multiple brands, establishing it as the flagship commercial program for Digital Customer Engagement. ZS has partnered with the client to provide them with innovation, process improvements, and automation support.
To bolster operations, ZS has also implemented a development capability on the Dataiku platform, complementing the existing operations with a ‘Dev + Ops’ setup which is lead to the onboarding of over 200 models on the Dataiku production environment, with a dedicated team of 90+ FTEs, including Data Engineers, Data Scientists, and Cloud experts. Challenges related to ML Ops capabilities, Pipeline orchestration, code versioning, and flow visualization were effectively addressed through the central Dataiku platform.
Business Solution:
Dataiku, a data science and machine learning platform, facilitates collaboration among analysts, data professionals, and scientists for various data projects. It offers a uniquely unified platform offering wide variety of data preparation, visualization, machine learning, and model deployment tools. Major challenges related to infra-computation for large-scale projects were addressed by transitioning from EMR to EKS clusters, optimizing Spark configurations, and enhancing Dataiku server performance.
Dataiku Upgrades and Optimization
Compute cluster change EMR to EKS on Dataiku:
- Improved Performance: Faster job execution enabled, compared to AWS EMR Spark Jobs. Scalability: Kubernetes cluster-auto scaler facilitates rapid instance scaling, three times faster than EMR.
- Cost Savings: EKS auto scales nodes when required, reducing the need for 24/7 Master and core nodes as observed in EMR.
- Version Support: DSS supports EMR versions 5.18 to 5.30; EMR versions above 5.30 (i.e., 5.31+, 6+ versions) is not compatible with the DSS.
- DS Jobs on EKS: DS jobs are already utilizing EKS for operations.
Job Optimization For Increased Performance:
Disabling Spark Dynamics Allocation:
- Issue: There were issues reported on jobs waiting for pods to launch.
- Solution: Disabled all the dynamic memory allocation from the spark configurations.
- Results: Issues reduced on the long running job in prod
Increasing Executors:
- Issue: Recipes that perform compute intensive tasks like dataset join etc., with the output produced are heavier, takes longer time to complete. Also, the data write speed from pod to s3 takes longer time and since this recipe has heavier datasets.
- Resolution: A unique spark configuration created involving high number of executors/instances to provide more resources and an addition in-code level to set the partition value to 40. This reduced the recipe runtime from 5+ hours to under 30 min.
Dataiku Server Optimization for Better Performance:
- Enabled Macros: Configured macros to kill idle Jupyter Notebooks after one hour.
- Kill Error Pods: Implemented scheduled cronjob to remove error pods, releasing cluster resources. Project Cleanup: Removed unnecessary projects from the environment to enhance Dataiku UI performance.
- Log Retention: Cleared Dataiku job logs with a retention period of 15 days
Cost Optimization:
- Cost of the EMR cluster will be cut down resulting in cost savings.
- Due to the optimization made on the job runtimes, the job takes lesser time to execute which directly effects the cluster node runtime and its cost.
Day-to-day Change:
Testimonials:
“I really appreciate the proactiveness of our ZS team to not only identify the issue but also providing a clear explanation to our key stakeholders on WHY this happened. Our ZS team also fixed this issue in record time. Our key stakeholders acknowledge this effort and the good discipline the ZS team has developed in resolving such issues. Please extend this appreciation to our ZS team.”
(Director, Machine Learning and Data Engineering)
“It has been a great partnership so far and looking forward to continuing this partnership to drive further value for the ZS team. I am particularly impressed with the progress made by the team in terms of technical understanding as well as brand specific knowledge in less than 3 months. We are happy with the overall work of the team.”
(Manager, Advance Analytics)
“Regarding “Standardization of Current Output generation process.” This will eliminate the tail end of the pipeline which is most error prone (and very time consuming). Thank you everyone for the hard work investigating and agreeing on a final output.”
(Principal Data Scientist)
Business Area Enhanced: Marketing/Sales/Customer Relationship Management
Use Case Stage: In Production
Value Generated:
Dollar values:
- The program values ~USD$9 million per year. From a client’s value perspective, we manage dynamic targeting for 15 brands producing ~USD$25 billion revenue i.e., assuming it increases the client’s top line by 2%, translation to ~USD$50 million.
- Cost Savings to the tune of $250k+/year based on multiple optimization techniques.
- Annualized Savings of ~400 effort hours for implemented Brands against ~360 hours in Pilot effort, translating to ~4000 hours for all Brands, when scaled across.
Runtime impact:
- Brand pipelines' run time reduced by 70% (16 hours vs 52 hours previously on EKS) and 50% (16 hours vs 30+ hours on EMR) – Dataiku backend Infra changes.
- Automated 35 manual touchpoints (25%) per brand per cycle. Approx. 2,400 Hours manual hours of effort saved annually (across brands).
- Reduction in pipeline reruns by 50% with improved quality control along with Optimized Run time of 17 hours in Test environment.
- Run time reduced by ~35 hours (Was 27 hours in EMR env. and 52 hours in EKS env ~70% reduction)
- 1.2 TB lesser data Processed or Shuffled during the run achieving ~60% reduction (2 TB to 800 GB)
- E2E effort saving is 30% of overall run time reduction anticipated.
- Approximately 4K manual effort saving across Dev and Ops.
- Successfully implemented an environment strategy for enhancements/test/prod environments to maintain versioning strategy.
Value Brought by Dataiku:
Without Dataiku process :
- Excel-based process
- High manual efforts
- QC-checks failure
- Error-prone results
With Dataiku Integration:
- Data Preparation and Integration: Dataiku offers intuitive visual tools for exploring, cleaning, transforming, and integrating data from various sources, including structured and unstructured data, streamlining complex data preparation tasks efficiently.
- Collaborative Environment: Dataiku provides a cross-collaborative workspace for data scientists, analysts, and business users to work together on projects. It also supports asset sharing, versioning, and knowledge sharing, promoting effective teamwork.
- One-Click Automation: With the help of Dataiku, we are able to run end-to-end complex pipeline without much manual intervention along with good quality QC checks automatically send out through mails.
- Machine Learning and Model Development: Dataiku equips users with a comprehensive set of tools for building, training, and evaluating machine learning models. It supports various algorithms and frameworks, enabling the integration of custom models into the platform.
- Governance and Security: With robust governance and security features, Dataiku ensures data privacy, compliance, and control. It offers user access control, data lineage tracking, and auditing capabilities, catering to enterprise-level security needs.
- Ecosystem Integration: Dataiku seamlessly integrates with diverse data storage systems, databases, data processing frameworks, and visualization tools. It allows users to work with their preferred technologies and effortlessly onboard datasets from different vendors.
- Automation and Orchestration: Dataiku facilitates easy replication and scaling across brands, leveraging old databases for efficient data crunching in a short time.
- Data Drift Modules: With Dataiku's built-in data drift plugin and visualization dashboard, calculating and monitoring data drift becomes simple, eliminating the need for custom code and reducing implementation efforts.
Value Type:
- Improve customer/employee satisfaction
- Increase revenue
- Reduce cost
- Save time
- Increase trust
Value Range: Dozens of millions of $