Air Canada - Post Campaign Analysis Automation
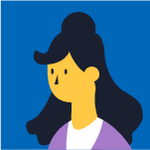
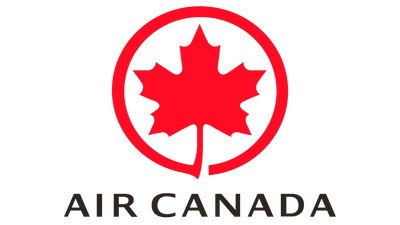
Team members:
Customer & Loyalty Analytics Team, with special mention to:
- Luis Miguel Caballero Chavez, Sr Data Analyst, Analytics - Route Marketing & Non Air Travel
- Adam Nagel, Manager, Analytics - Retail & LCM
- Luc Gagnon, Director, Analytics- Travel Marketing & Loyalty Spend
Country: Canada
Organization: Air Canada
Air Canada is the largest provider of scheduled passenger services in the Canadian market as well as in the Canada-U.S. transborder and the international markets to and from Canada. We carried more than 37 million customers in 2022, to 185 direct destination world-wide. This represents close to 1,000 flights daily. The company grew to close to 36,000 active employees in 2022.
Aeroplan’s membership has already exceeded its 7M active members target. The Customer & Loyalty Analytics team has the privilege to aim at better understanding these customers and Aeroplan Member behaviours so that we can serve them better!
Awards Categories:
- Best Acceleration Use Case
- Best Data Democratization Program
- Best Approach for Building Trust in AI
- Best ROI Story
Business Challenge:
The Customer and Loyalty Analytics Team works closely with our Marketing Team in order to design and run marketing campaigns.
After each campaign, there is a need to understand its performance, so that we can repeat what works (and not repeat what doesn’t work) in subsequent campaigns. Our Post Campaign Analysis (PCA) assesses the campaign performance by looking at multiple datapoints like communication metrics (Email open, Email clicks, Website Banner Impressions and Clicks, Paid Media Impressions & Clicks, Registration, Flight Searches), Conversion Metrics (Flight Bookings, Flight Revenues, Points Earned, Credit Card Applications, etc.), Incrementality (Booking & Revenue), and Marketing Channel Attribution.
Post-campaign analysis presented the following challenges:
- Data was scattered across different places, into multiple warehouses.
- All analyses were performed on an ad hoc basis, as there was no way to automate and schedule processes.
- PCAS were not timely enough to deliver insights right after a campaign ends, which doesn’t allow us to apply learnings on the next campaign.
- We also didn't have the capacity to perform PCA manually on all significant campaigns, therefore lacked valuable data points to build upon success.
Business Solution:
We first solved for data centralization into one place with Snowflake. Then, we leveraged Dataiku’s visual flows and DataOps to automate and schedule the PCA process.
The C&L Analytics team generalized (parametrized) the processes so that our marketing stakeholders would only need to provide us with the campaign parameters (e.g. booking dates, travel dates, origin & destination, campaign project number, promo codes, registration codes, etc.) that are required to run a PCA process and started running the automated PCAs in batches, instead of manual bespoke ad hoc analysis.
Once processes are run, all outputs are pushed to PowerBI in one single dashboard for consumption by our Marketing Stakeholders.
Day-to-day Change:
Our Marketing Stakeholders have quicker access to more PCA insights (on all campaigns) and can make better and quicker decisions to continuously increase performance over time.
By automating the tasks, Data Scientists can invest more time on “what we don't yet know how to do”, aiming at maximizing the use of their time on added value efforts to expand our ability to understand our customers and predict their behaviour.
Business Area Enhanced: Marketing/Sales/Customer Relationship Management
Use Case Stage: Proof of Concept
Value Generated:
- From 2 weeks elapsed time for 1 PCA to running 12 PCA in 3.5 hours.
- Resource utilization improvements of 96% - With more than 200 campaigns or potential PCAs per year, it would have cost over $400,000 in avg data scientist salary to produce all these insights as opposed to a fixed cost of ~$15,000 with the new automated PCA process. This represents an opportunity cost of 96% improvement over the prior unscalable manual solution.
- Marketing effectiveness revenue gains - We also know that applying these valuable insights quicker and on more campaigns will have an impact on future revenues, which is harder to assess at this point. This value is incremental to the resource utilization improvements and is expected to significantly exceed these.
- Fulfilled and eliminated ~90% of Campaign PCA requests. Only deep dives on select campaigns remain.
- No more backlog - PCA available for all campaigns, as opposed to a subset of the most important ones.
- Quicker access to insights, which allow continuous learning and improvement of performance at regular intervals (rather than static view).
- Opportunity cost saving: Data Scientists now have more time to invest on more added value projects!
Value Brought by Dataiku:
Dataiku enabled the orchestration of the processes, allowing us to automate, create the flow and schedule our processes as opposed to have to manually re-code and treat these projects as ad hoc analysis, which has reduced analyst’s efforts to a few hours 1 day a week. The seamless integration with Snowflake (to easily access the data) and PowerBI (to easily showcase the outputs) also helped streamline the whole process.
Value Type:
- Increase revenue
- Save time
Value Range: Thousands of $