AWR Automotive – Predicting Customer Lifetime Value
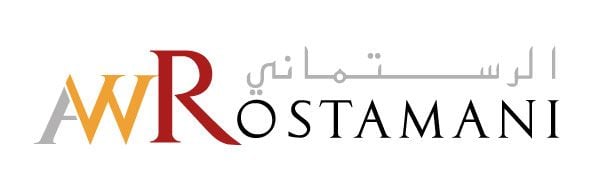
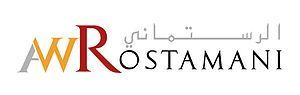
Team members:
- Sethuramalingam Subramaniam, Senior Data Scientist
- Dr. Mani Abedini, Data Science Manager
- Sebastian Samuel, Chief Information Office
- Abhiruchi Tiwary, Lifecycle manager
Country: United Arab Emirates
Organization: AWR Automotive
The AW Rostamani Group, also known as “AWR”, is a privately held company has a diverse portfolio of businesses operating across various industries, including Automotive, Real estate, Ventures & Investments. Driven by the purpose of enriching lives, we have grown into a conglomerate of many companies with presence in seven diverse sectors and strive to help shape a better world with our exceptional products and services.
Automotive: The largest Business unit of the group and the leading automobile dealer in the region, operates several automobile dealerships, representing brands such as Nissan, Renault, Infiniti.
Real Estate: The AWRostamani Group is involved in real estate development and property management. They have been engaged in various residential and commercial projects in Dubai.
Ventures: Our ventures arm include BCD Travel, AWR Lifestyle, KAR Freight and forwarding, AWR Lumina and AWR Logistics.
Investments: AWRostamani Group has a large and diversified local and global investment portfolio, with a primary focus on capital appreciation and dividend yield.
Awards Categories:
- Best Acceleration Use Case
- Best Moonshot Use Case
- Best Data Democratization Program
- Best ROI Story
Business Challenge:
Acquiring new customers is typically more expensive than retaining existing. As customer behaviours evolve over time, with increasing competition in our market, retention of loyal customers is a Herculean task for our business. Yet running customer retention campaigns on inappropriate contacts will lead to further revenue loss.
Therefore, it is quite imperative to identify high-value customers and nurture them to maintain a long-lasting relationship. Several case studies have proved that any investment for retaining long-term customers is more cost-effective and yields efficient results.
We proudly stand as one of Dubai's premier automobile dealers, renowned for representing the most sought-after brands in this thriving region. We have customers with varied demographic profiles, visiting our showrooms for retail purchase of new or used cars daily. We also cater to all customers who service their vehicles in our workshops spread across the Northern UAE region. Once a customer purchases a vehicle from us, we nurture the customer as part of our after-sales business to service the vehicle from any of our workshops. As customer behaviors vary between sales and after-sales, it’s not possible to compute the customer lifetime value (CLTV) of a customer using a single model.
To address the above challenges, we decided to build a unified model for predicting CLTV, to accurately calculate customer satisfaction, loyalty, and long-term profitability.
Business Solution:
We have chosen Dataiku as our platform for the use case starting from feature engineering until deployment. The versatile platform empowered us to build the end-to-end use case by offering data integration, preparation, machine learning capabilities, visual analysis, automated training, deployment & redeployment based on best accuracy scores, etc.
Our approach is inspired by Dataiku’s CLTV prediction as part of their pre-canned industry solutions.
As part of our CLTV prediction project, we envisaged to explore and solve the following business problems:
- Predict CLTV from sales and after-sales / service data separately
- Compute the RFM (Recency, Frequency and Monetary) segments based on sales data
- Compute the RFM segments based on services data
- Create customer segments based on customers’ demographics
- Computer overall customer CLTV
- Predict the remaining potential number of years in business for customers
The first problem we tried to solve is to forecast the CLTV value for sales and after-sales separately as there are clear differences in terms of monetary value and frequency between how often a customer purchases or upgrades their car vs. how often they service their car. So, based on our years of data from sales and after-sales, we built parallel pipelines to pre-process sales and service data from previous years, then merge them with the vehicle ownership details of each customer.
As services are carried at the vehicle level, we tried to forecast the service CLTV at the vehicle level, whereas sales CLTV is estimated at the customer level. Through merging with vehicle ownership data, we can sum up the service CLTV for customers based on the vehicles owned.
The RFM segments are calculated based on quantile-based clustering of recency, frequency and monetary values at both sales and service levels separately.
Customers’ demographics like age, nationality, gender, number of cars owned, etc. are considered as features captured at the customer level and we clustered them using K-means.
The RFM segments’ scores, customers cluster ids, sales and service transactions data were finally used to build regression-based models to predict sales and service CLTV scores respectively.
We achieved an R2 score accuracy of more than 0.84 for service and a R2 score accuracy of more than 0.9 on the sales data.
The predicted sales and services CLTV scores of a customer are finally added to return a single estimate CLTV value for every customer.
We also built dashboards with Dataiku to track the influence of the various scores at each level to the overall CLTV scores. Business users use these dashboards for monitoring.
Dataiku helped us build this complete architecture from the ground up. We were able to completely build this solution using built-in visual recipes for most parts of the pipelines – from pre-processing recipes to visual recipes for unsupervised learning like clustering, as well as for building supervised learning-based prediction of the final CLTV scores.
As CLTV scores are not actionable on their own, we plan to leverage the estimated CLTV score as an additional attribute of a customer in upcoming data science projects.
Key capabilities from Dataiku on this journey include:
- Best compute capabilities for large datasets using Kubernetes
- ETL Pipeline development on the go
- Feature engineering at fingertips
- Version control
- Rapid prototyping and dashboard reporting
- Shareability of reports and project across teams and business users
Business Area Enhanced: Supply Chain/Supplier Management/Service Delivery
Use Case Stage: In Production
Value Generated
The predictive analytics use case implemented in collaboration with Dataiku has generated accurate prediction of CLTV scores both for the AWR Sales and for the AWR Service Departments.
We plan to utilize this information to better target our high-value customers with relevant offers via our marketing campaigns. For example, business can optimize the discount budgets towards high value customers (based on CLTV value) to make ensure customer satisfaction a return of investment.
The model is directly enriching customer 360, better understanding of customer behaviour, personalizing offers, improving customer satisfaction, which lead to reducing customer churn, increasing revenue of sales and service, etc.
The project has also helped us minimize generic targeting of customers with irrelevant offers and promotions.
Value Brought by Dataiku:
- End to end integrated data science platform
- Streamlined model maintenance and deployment/redeployment at scale using Kubernetes
- Enhanced team collaboration and data utilization
- Effective, rapid dashboarding and reporting
Overall, the CLTV prediction use case has empowered our Sales and Services Departments to take a data-driven approach to customer retention. By leveraging data insights and predictions, the business can deliver targeted, personalized campaigns to enhance customer loyalty and ultimately drive long-term profitability.
Value Type:
- Improve customer/employee satisfaction
- Increase revenue
- Reduce cost