Travis Perkins - Fraud Detection in the Construction Industry
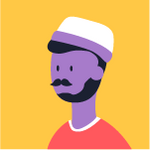
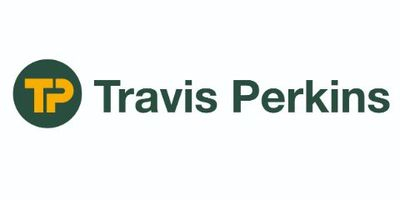
Name: George Brookes, Data Scientist
Country: United Kingdom
Organization: Travis Perkins PLC
Travis Perkins plc is the UK’s leading supplier of materials and equipment to the building, construction and home improvement markets, and operates market leading businesses, including Travis Perkins Builders’ Merchants, Toolstation and a number of specialists in the civil engineering, plumbing, heating and insulation markets, such as Keyline, BSS and CCF. With annual revenues of £5bn, the Group employs 16,000 colleagues across the UK, but also in France and Benelux through Toolstation. The company has a proud heritage that spans over 200 years.
Awards Categories:
- Best Data Democratization Program
- Best ROI Story
Business Challenge:
Because of the nature of the construction sector where contractors may only get full payment for their work once the job is completed, Travis Perkins Plc offers trade credit accounts. This allows our customers to purchase materials and tools and pay later, within a specified period of time.
Whilst there are many benefits of using a trade credit account, such as helping with seasonal fluctuations in sales, there are also associated risks. One of them is fraud. fraudsters may attempt to set up and use credit accounts that they have no intention in paying the balance off on.
To set a credit account up the fraudster would have to provide personal information. This can be accurate information about themselves but more often it is the information of some unsuspecting person. Travis Perkins Fraud team were able to analyse fraudulent cases after they have occurred and take action on a case-by-case basis. The analysis process was manual and resource-intensive.
Previously there were no data capabilities developed to help identify orders that could be fraudulent before they were sent out. Sometimes the first time that we would know that an order was fraudulent would be when a bank would send the business a credit chargeback of the value of an order that a genuine customer said that their card had been used without their knowledge. In these cases we were essentially being changed twice, once for the value of the goods and again for the chargeback.
Whilst the Fraud team relied on manual processes for fraud detection, they built a history of previous fraudulent cases. This history was the starting point for Fraud & Data Science team collaboration. The thought process was that we could use this information to flag any credit accounts placing orders that had matching information with a credit account that had previously been used for fraud.
Business Solution:
Travis Perkins Plc has a large customer base. In order to check the history of known fraud cases against our open orders data available in the data lake, we needed to do many-to-many comparisons across a number of fields. This complex logic and the need for the cross-team collaboration was why we chose to develop our solutions in the Dataiku platform.
In Dataiku, we were able to read in the current open orders and also the information on the previous fraud cases. We made use of the distinct operations to reduce the complexity of the data we needed to analyse.
The built in python capabilities helped us write elaborate scripts to do our many-to-many comparisons. This first step would give us the reason for open orders being flagged, for example, phone numbers matching to previously confirmed fraud cases.
The next step was to present this information as 3 reports:
- Data of the open order and the reason that it was being flagged.
- Aggregation of the reasons for flagging, as multiple open orders could be being flagged for the same reasons and the Fraud team would want to know reasons with the most open orders and the reasons that had the most monetary value.
- History of fraud cases that were applicable to the flagged reasons.
The team that developed this project consisted of a data scientist and two subject matter experts from the fraud team. Dataiku was the best way we could get this done because of its explainability not only amongst non technical members of the development but also when it came to explaining the solution to the wider business.
The combination of the business knowledge brought by the fraud team and the technical skills provided by the data science team as well as the collaborative approach that the Dataiku platform allows for gave us the opportunity to provide a fix to an expensive area of our business.
Day-to-day Change:
Now we are able to proactively identify possible fraud cases rather than reactively identify patterns in the data. Another value that has been brought by this solution is the Fraud team can now work in a more efficient way. Being able to prioritise their time spent investigating all possible fraud cases down to just the few that the solution identifies.
Business Area Enhanced: Other - Fraud
Use Case Stage: In Production
Value Generated:
There was no specific investment for this project other than the Dataiku platform which has been budgeted for and the time of the three colleagues who lead the development. So this means the return on investment of £20,000 in the first five days was purely profit. Or more accurately the solution saved the business £20k that it would have lost otherwise.
Value Brought by Dataiku:
- Data preparation: Dataiku made it easier for subject matter experts from the Fraud team to explain their manual process to the data scientist. In turn, data scientist was able to clean and prepare data in collaboration with the fraud team due to the visual capabilities of the Dataiku platform
- Model review & deployment: it was easy to explain how the new process works and how many lines of data it goes through before arriving at final results. This helped to build trust with the Fraud team as they could review every step in the process. The Dataiku platform made it easy to automate the production model whilst retaining full flexibility for further developments
- AI/ML capabilities: having proven the added-value of using Dataiku platform, the Fraud team is now open to ML/AI capabilities and exploring new ways of detecting fraud.
Value Type:
- Increase revenue
- Reduce cost
- Other - More efficient processes
Value Range: Thousands of $
Comments
-
Incredible stuff here. Great work George!