Talent Access - Creating a Dashboard to Analyze Stock Price Trends and Market Sentiment
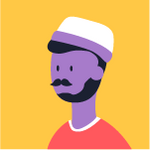
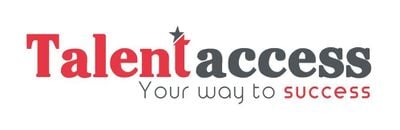
Name: Naoufal Rahali
Title: Managing Partner
Country: Morocco
Organization: Talent Access
Talent Access is an AI/Data Consulting company working with organizations, especially in the public sector, to develop data capital.
Awards Categories:
- Best Moonshot Use Case
Business Challenge:
Getting quick intuition about market sentiment related to a stock based on multi-source news scrapping (local news websites, paid providers, stock exchange platform). On the one hand, the client claimed to be spending too much time tracking news about targeted stocks. On the other hand, the decision is based mainly on traditional fundamental analysis interpreted by humans (either internal stakeholders or external consultants), but no data-driven approach was applied to get the "right" decision.
This process is performed continuously as the client has to make decisions about selling and buying on a weekly basis.
Business Solution:
Since the client was conducting a pilot, they wanted to have a quick idea about the opportunities that AI/ML could offer in portfolio management. With Dataiku, we had the possibility to iterate quickly and show in a progressive way the possible deliverables.
There were two main projects: 1) Data collection and preparation and 2) ML model building.
Data collection involved the daily collection of stock prices for a targeted portfolio, as well as the collection of news from different media that the team typically checked daily in order to have an idea about any targeted companies (new projects, organization change, certifications, etc...).
For ML model building, we created the first model based on GPT3 from OpenAI that gives news sentiment (positive, negative, or neutral). We also created another customized model that tries to find any relation between past news and stock price trends, then gives predictions based on the last news collected.
We were also able to get a time series model prediction as an intuition for possible stock price trends (based on historical data, which is based on the hypothesis of possible autoregression). All model outputs were consolidated on one dashboard that the team could interact with and also receive by email.
Day-to-day Change:
The team gained back time that was typically spent checking news from different sources, then analyzing it to try to predict the potential impact on stock prices. In addition, the team was able to have a dashboard that gives quick intuition about market sentiment related to a given stock.
Business Area: Financial Services Specific
Use Case Stage: Proof of Concept
Value Generated:
A team made up of two full-time financial analysts was dedicating 25% of their time to keeping themselves updated about the targeted stocks, with the risk of missing information. The POC made it possible to better use this time for more in-depth analysis based on the dashboard that was built with Dataiku, as the team managed to track all news sources in one space that offered visual sentiment analysis on the go.
Value Brought by Dataiku:
Mainly the speed and agility to experiment with the client's request, as the pilot was planned for 90 days. During this period, there were many iterations (2-3 weeks) with a delivered value starting from news collection, to data transformation and preparation, to model experimentation, and, finally, to dashboard building.
Dataiku comes with a set of tools that makes it easier to accelerate this process either for web scrapping or text preparation. For model construction, there also was a large set of prebuilt (time series model) and also the plugin to adapt GPT3 quickly for the specific use case.
All the process was shared with the team on the go, and feedback could be retrieved instantly. What's more, the process of modeling was transparent and explainable for all stakeholders, which is critical for making decisions.
Value Type: Save time
Value Range: Thousands of $