Javier Coronado Blázquez - Leveraging MLOps to Study the Spanish Real Estate Market and Estimate Mar
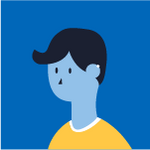
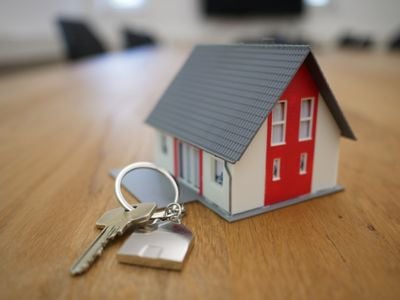
Name: Javier Coronado Blázquez
Title: Data Scientist, PhD in Theoretical Physics and Cosmology
Country: Spain
Awards Categories:
- Best Positive Impact Use Case
Business Challenge:
Following COVID-19 and the war in Ukraine, the world has experienced very high inflation and rising prices. In Spain, this has been particularly true for the real estate market, where, since 2017, the housing price has been rising, with the exception of 2020. In big cities such as Madrid, the price rise has been so significant it has become difficult to estimate whether a particular property is under or overpriced with respect to the rest of the market.
Taking an ML-based approach, we aimed to understand the main characteristics of each property (e.g., area, number of rooms, real estate agent or particular vendor, neighborhood characteristics) affecting the price to 1) determine whether the listing price is reasonable or not, and 2) highlight the principal points affecting it, thus identifying the most useful negotiation levers.
Business Solution:
This was a personal project. The final aim of it would be to study the Spanish real estate market and use MLOps to extract data on a monthly basis from the main web portals and study the trends based on several key factors.
The project's current state is a proof of concept (PoC) made by one single user, in which housing data for the whole Madrid metropolitan area is scraped from Idealista (the leading Spanish real estate ad listing site). This offers around 20,000 different properties, covering all price ranges and neighborhoods. Additionally, data from the Madrid town hall regarding cadastral reference aggregated by neighborhoods is used to enrich the dataset.
With Dataiku, preprocessing is performed to standardize and extract the different information. With automatic dashboard generation, several key factors in the statistical distributions are studied to uncover patterns. Then, several algorithms are tested in a regression to obtain the estimated retail price. Finally, the output is studied to understand the main factors driving the price up or down, as well as using the interactive simulator to compute the price for a new house.
This tool could also be used by real estate agents when estimating the property's market value.
Use Case Stage: Proof of Concept
Value Generated:
With this PoC, any user interested in Madrid's real estate could quickly feed the pipeline with a putative property and obtain a price estimation, to know how under or overpriced it is with respect to the rest of the real estate market.
In future phases, the pipeline would automatically ingest data with a monthly or quarter periodicity from several web portals, and a user who is inexperienced in either ML or the Spanish real estate market could immediately obtain an estimation of the price using the interactive simulator. This is especially useful if an individual is to move to a new city that is largely unknown to them. In this case, they would introduce some fixed variables (e.g., number of rooms, floor area) and play with the rest of them to see if the estimated price is within their budget.
The current ML pipeline, with 20,000 properties listed in the Madrid metropolitan area as of January 2023, presents excellent results, with an R2 score of about 0.9 and a Pearson correlation of 0.95. This provides encouragement to keep on developing and scaling this project to include more cities and automatic updates. It would also allow for a price estimation in different epochs, drawing the evolution of price with time for a single property, its neighborhood, its city, etc.
Value Brought by Dataiku:
Dataiku provided a very intuitive way to deploy all the necessary steps in this pipeline and the possibility to scale it up automatically, provided more data was ingested. The automatic dashboards provide immediate insights into the data, and the interactive tool makes it easy for new, inexperienced users (either in ML, real estate, or both) to compute a reference price for their house of interest.
Value Type:
- Improve customer/employee satisfaction
- Save time