Unilever - Creating Data-driven Product Ideas Based Exclusively on Consumer Wants and Needs
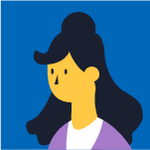
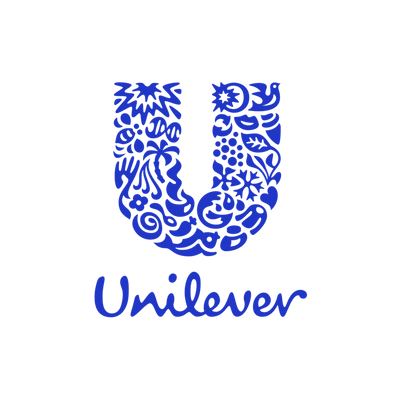
Name:
Linda Hoeberigs, Sr Manager, Data Science & AI – CMI PDC Labs
Victor Ignatiuc, Sr. Data Scientist – CMI PDC Labs
Sanjeev Chandran, Sr. Data Scientist – CMI PDC Labs
Pierluigi Costanzo, Sr. Data Scientist – CMI PDC
Grzegorz Strelczuk, Data Engineer – CMI PDC Labs
Vasilis Tsiadis, Service Delivery Lead – CMI PDC Labs
Sophie Bull, Change and Comms Analyst – CMI PDC
Chandan Agarwal, CMI PC Director – PDC and Advanced Analytics
Chhavi Agarwal, CMI PDC India Lead
Alfred Barretto, CMI PDC India Analyst
Christi Kobierecka, CMI PDC Home Care Lead
Patrick van Hauwe, CMI PDC BPC Lead
Sandra Morgan, CMI PDC PC Lead
Freek Vrijhof, CMI PDC Foods Analyst
Craig Steward-Thompson, UniOps Data Architect
Diwaker Tandon, UniOps Data Engineer
Country: Netherlands
Organization: Unilever
Unilever is one of the world’s leading suppliers of Beauty & Personal Care, Home Care, and Foods & Refreshment products, with sales in over 190 countries and products used by 3.4 billion people every day. We have 148,000 employees and generated sales of €52.4 billion in 2021. Over half of our footprint is in developing and emerging markets. We have around 400 brands found in homes all over the world – including iconic global brands like Dove, Lifebuoy, Knorr, Magnum, OMO, and Surf; and other brands such as Love Beauty & Planet, Hourglass, Seventh Generation, and The Vegetarian Butcher.
Awards Categories:
- Value at Scale
- Moonshot Pioneer(s)
- Most Impactful Transformation Story
Business Challenge:
Unilever has a rigorous idea process for new product development, which covers everything from trend detection to idea screening.
As a global consumer goods company with over 400 brands in 190 countries, there are a lot of category and regional nuances to the innovation process. The Consumer & Market Insight team works globally and locally to streamline the said process, ensuring both local and category nuances are taken into consideration.
Because Unilever has a vast and varied category and market footprint, each business group (product category) and unit (countries) have a unique set of challenges that need to be considered during innovation and product development, which meant there was an opportunity for our innovation process to become faster, more automated, more agile and more consumer-driven, 100% based on data use.
As an organization, we needed to become more nimble to keep Unilever brands at the forefront of upcoming market disruptions through new product trends and serving consumers where their needs and wants are.
Business Solution:
To accomplish this and combat the associated challenges, the CMI People Data Centre was tasked with creating an automated, end-to-end idea spotting, screening, and sizing solution. It would move from early trend detection to validated concepts, resulting in products driven purely by consumer wants and needs in a fully automated manner. This solution had to accommodate the nuances of local markets and the respective brands while delivering a cohesive global tool that could be used by all business groups.
The solution was to integrate consumer wants and needs, as well as product and market information. All of this is only available in highly unstructured online data. It needs to be both clustered and used in machine and deep learning algorithms to mimic consumer wants and needs and truly automate the process, free from human input.
The complexity of this data science problem led us to a mix of data collection methods, all of which were facilitated in the Dataiku platform. Wrangling these datasets, which included seven billion ratings and reviews, two billion data points of consumer searches, and a plethora of social media data, we used the Dataiku flow. While doing so, we enriched our data with Unilever propriety concepts, which we predicted using deep learning. In this data wrangling, we assessed over 100 billion potential product ideas to arrive at 500 million viable new ideas (high demand, low product availability white spots).
Using various NLP solutions, we also assessed the uniqueness of these ideas versus everything on the market. Once ready, we build various machine learning solutions to understand which of these ideas best fit consumer preferences, based on historical data of innovation success and failures and their performance on various metrics during the pre-launch period. Following that, we send the best-performing ideas to consumer testing. The output goes into our ML solution for sizing the product ideas (sales forecasting).
Business area enhanced: Analytics/Strategy/Marketing/Sales/Customer Relationship Management/Product & Service Development/Product Innovation
Use case stage: In Production
Value Generated:
Rather than assessing as few as 100 new product ideas over the course of eight months, Unilever now has the ability to consider 500 million product ideas over the span of four to 14 days.
Human input hours have been reduced from three months of full time effort to three days of spot-checking our results for brand safety to exclude areas where Unilever doesn’t play despite there being consumer demand.
Furthermore, this solution ensures that Unilever’s new product ideas are truly data-driven by basing them exclusively on consumer wants and needs that come through in their data.
This solution frees up our brand managers and marketers to identify newer ways of delighting our consumers and customers.
Our ideas have also performed well in consumer testing.
There are currently 41 Athena products in the market, and early signals show that the majority of them have beaten their business cases, which means their in-market performance is stronger than expected.
We have examples of products that went from Athena idea to in market in less than 100 days and delivered double the business case.
In terms of future products, the 70 Athena products which are currently in the development stage, are projected at a business case twice the average size of a Unilever innovation.
Value Brought by Dataiku:
Dataiku helped us to systemically and efficiently analyze 100s of Gigabytes (sometimes terabytes) worth of text data. While doing this analysis, we’re able to pick various elements (datasets, recipes, models) from other projects to avoid duplication.
Through the visual interface, both our market research analysts, who input category knowledge, and data engineers and scientists, who build the pipeline, could work together on this project efficiently.
Through its flexibility, we experimented with different predictive algorithms, clustering techniques, and embeddings to find the most effective ones for each stage. The visual deep learning made these methods much more accessible to us than they would have been otherwise, saving a lot of time.
Value type:
- Increase revenue
- Reduce cost
- Save time
- Putting the consumer at the heart of the innovation process
Value range: Tens of millions of $ and above