Using Tensorflow in a Custom Python Model.
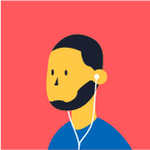
UserBird
Dataiker, Alpha Tester Posts: 535 Dataiker
To use the tensorflow in the custom python model, the code needs to provide the methods fit() and predict(), like SK-Learn.
The code below is the code that I think I need to use.
import tensorflow as tf
# Specify that all features have real-value data
feature_columns = [tf.contrib.layers.real_valued_column("", dimension=4)]
# Build 3 layer DNN with 10, 20, 10 units respectively.
classifier = tf.contrib.learn.DNNClassifier(feature_columns=feature_columns,
hidden_units=[10, 20, 10],
n_classes=3,
model_dir="/tmp/iris_model")
# Define the training inputs
def get_train_inputs():
x = tf.constant(training_set.data)
y = tf.constant(training_set.target)
return x, y
# Fit model.
classifier.fit(input_fn=get_train_inputs, steps=2000)
What I think the problem is, I need to change the input into tf.constant and send them to the fit method.
But I have no idea how the data is retrieved or the variable name that is used in the fit method.
Does anyone have a sample code, or know the walk away round?
I am new to python, ML, DDS everything so please help.
Tagged:
Answers
-
Hello,
Interesting question. The issue here is that tensorflow models cannot be serialized through pickle as weight matrices are saved to external files. In theory you can build a wrapper around keras classifier (with tensorflow backend) to make it pickleable. It works by saving weight matrices to memory. You can have a look at https://pypi.python.org/pypi/keras-pickle-wrapper/1.0.3. This is untested, so let us know if it works.
Having said that, you can perfectly use tensorflow in a Python recipe or notebook, outside of the "Custom model" interface.
Cheers,
Alex