SLB - Predictive Maintenance to Improve Reliability of Drilling Services
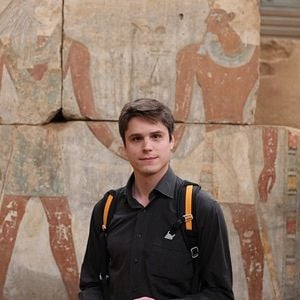
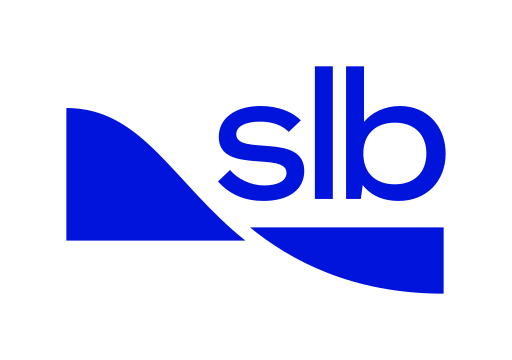
Name:
Valerian Guillot
Darwin Amaya
Eimund Liland
Hafiza Had
Kiran Chand Puthiyottil
Michael Love
Country: France
Organization: SLB
SLB is a technology company that partners with customers to access energy. Our people, representing over 160 nationalities, are providing leading digital solutions and deploying innovative technologies to enable performance and sustainability for the global energy industry.
Awards Categories:
- Value at Scale
- Moonshot Pioneer(s)
Business Challenge:
SLB (SLB: NYSE) is a technology company that partners with customers to access energy. One of the critical components that SLB provides is directional drilling services, which are allowing customers to land a well trajectory in the optimum location. Those directional drilling services are provided using a rotary steerable system (RSS).
The rotary steerable system is being used under very hard environmental conditions (shocks, vibrations, temperature), and this may cause equipment to fail. The consequences of such equipment failure are severe and will cause the drilling bottom hole assembly to become unable to follow the planned trajectory. This generates very costly remediation work.
In addition to the failure mechanism described above, there are multiple other failure modes and mechanisms that may occur in a Rotary Steerable System.
To ensure that SLB’s operations are performant & reliable, over the past 15 years, LB has implemented a strategy of instrumenting the drilling equipment and collecting systematically the data.
This systematic data collection has led to multiple terabytes of times series data, contextualized with meta information about drilling context, and maintenance actions.
This case study will focus on one of the failure mechanisms, when the rotary steerable system hinge pin is breaking.
The steering of the well trajectory is done by actuating the tool pads, so that they apply force on the geological formation, in the direction opposite to the desired direction while the rotary steerable tool is rotating. The force on the formation is applied by moving a pad, which is rotating along a hinge ping, on the side of the tool.
The business challenge is therefore the necessity to reduce very high impact equipment failure, by implementing preventive maintenance actions, leveraging the opportunity of having a huge pool of data to learn from.
Business Solution:
The essential element of improving equipment reliability is making sure that the manufacturing engineers, maintenance engineers, service quality engineers have an easy access to the data. Since they are the individuals that are the most familiar with the tool, and the way it is being used, they are the people that are the most likely to build the most meaningful features to predict the equipment failure.
We have therefore:
- Connected Dataiku to the aggregated data, through direct connections, and Dataiku plugins
- Trained engineers (maintenance, sustaining) on how-to
From there, with occasional data science guidance, the sustaining & maintenance teams have been engineering Dataiku flows, and models to best characterize and predict when the hinge pin would break.
The next problem to solve, was being able to yield actual value from the model, by utilizing the insights.
The two main avenues we have been using to utilize the model are:
- Business Intelligence dashboard, where the model is being applied on the equipment inventory, to flag at risk equipment
- Including the Dataiku trained model within a tool diagnosing application, so that the equipment at risk is flagged when the tool is being maintained.
Empowering our maintenance & sustaining technical experts in Dataiku has been key in understanding the usage patterns of SLB drilling equipment. We are also now taking a systematic approach at looking at data to help us improve the reliability of SLB’s operations with our customers.
Business Area: Analytics
Use Case Stage: In Production
Value Generated:
For the failure mechanisms that are predictable with the data available, applying the models in production reduces the likelihood of failure by a factor of 5x, thus incrementally improving the reliability of the directional drilling services provided to SLB's customers.
Value Brought by Dataiku:
SLB strongly believes in empowering domain experts (Manufacturing, Sustaining, Service Quality, ...) with data. Dataiku, and its ability to support domain driven extensions via plugins has been a key component to the success of the data-driven initiatives.
Value Type: Increase revenue, Save time, Improve customer/employee satisfaction