Creatring a custom scoring metric
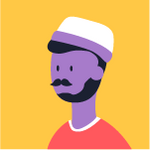
Hello @Alex_Combessie
,
I want to code a custom scoring function for scikit-learn's average-precision-score. It's a for a Keras image binary classification model. I'm not familiar with how the Dataiku API works, but I want to pass the array dimensions to y_valid and y_pred.
Here's what I have:
from sklearn.metrics import average_precision_score
def score(y_valid, y_pred):
"""
Custom scoring function.
Must return a float quantifying the estimator prediction quality.
- y_valid is a pandas Series
- y_pred is a numpy ndarray with shape:
- (nb_records,) for regression problems and classification problems
where 'needs probas' (see below) is false
(for classification, the values are the numeric class indexes)
- (nb_records, nb_classes) for classification problems where
'needs probas' is true
- [optional] X_valid is a dataframe with shape (nb_records, nb_input_features)
- [optional] sample_weight is a numpy ndarray with shape (nb_records,)
NB: this option requires a variable set as "Sample weights"
"""
average_precision = average_precision_score(y_valid, y_pred)
return average_precision
Best Answer
-
Hi,
That seems to be a good piece of code to start with. Are you encountering an error when training? If so, could you post the error message, please?
Hope it helps,
Alex
Answers
-
Works great, thanks for verifying