Credit Suisse Group AG - Designing Scalable ML Solutions to Increase Sales Leads and Grow Revenue
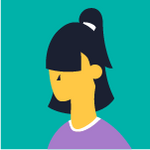
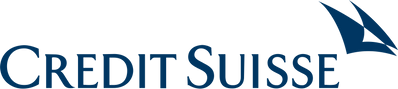
Names:
Marco Menner, Senior Data Scientist, on behalf of Credit Suisse – Swiss Bank Advanced & Sales Analytics:
Carsten Staas, Head of Mgmt & Business Information Analytics
Beat Huggler, Head of Advanced & Sales Analytics
Detlef Repplinger, Head of Data Science and Analytics.–Team of 6 Data Scientists & Engineers
Grahame Adderley, Head of LBM & Analytics – Team of 3 Business Information Analysts
Country: Switzerland
Organization: Credit Suisse Group AG
Credit Suisse is one of the world's leading financial services providers founded in 1856 and based in Switzerland. Our strategy builds on Credit Suisse's core strengths: its position as a leading wealth manager, its specialist investment banking capabilities, and its strong presence in our home market of Switzerland. As of mid-2022 Credit Suisse employs approximately 51,030 people.
Awards Categories:
- Value at Scale
- Most Impactful Transformation Story
- Most Extraordinary AI Maker(s)
Business Challenge:
Introducing machine learning solutions at scale in a large bank with measurable business impact has several challenges. Overcoming the following three challenges were key to our success:
First, to make use of and consolidate the complex data landscape across the bank, which comprises a large variety of insightful data sources spread across the bank at petabyte scale. Our mandate is to utilize such data to generate measurable impact from analytics and machine learning by improving sales activities and customer satisfaction in line with the current regulatory framework.
Second, to manage a large and ever-increasing number of machine learning models and business rules in production by utilizing thousands of data sources for a complete coverage of all customer relationships within various business areas. Here we need to be able to manage our production cycle while keeping the overview.
Third, to closely collaborate with number of different stakeholders, such as sales management, sales agents, product experts, channels owners and IT in a productive way and making sure that our analytics solutions are actionable, transparent, interactive with a high adoption by sales agents and clients.
Our mission is to deliver our analytics solutions at scale with a relatively small but effective team of Data Scientist in the business. To be able to deliver our mission and manage our large number of ML models in production, while at the same time accelerating new developments, a powerful data science workbench such as Dataiku became essential.
Business Solution:
We started our journey by building first ML models in Python to deliver sales opportunities to private banking sales agents in 2016. In 2017, we delivered those opportunities to a new CRM system with better activity and impact tracking as well as a feedback loop. We soon reached a point at which a modern data science workbench became essential to reduce development cycles and to manage our increasing data and model pipeline more professionally.
Later in 2017 we selected Dataiku as our data science workbench as an early adopter within Credit Suisse. We chose Dataiku since it provided the best value for price compared to other platform providers and was the strongest vendor for offering operationalization functionalities via the automation node. Choosing Dataiku early on demonstrated the amount of trust we put into the Dataiku platform already in the beginning. Working with Dataiku allowed our data scientists and data engineers (currently comprising eight users) to extend the scope to also covering corporate clients and leveraging ML insights for first online campaigns.
Dataiku allowed us to conveniently migrate our existing pipelines in Python and to increase our model scope and frequency of our sales lead deliveries. We were able to schedule our complex data pipeline and our model runs in production much more efficiently by utilizing the large variety of coding recipes and data source types covered on Dataiku.
Dataiku enabled us to onboard a team of business information analysts, enabling query scheduling for more rules-based data insights. We were also able to unlock a new form of collaboration with a team of business users from sales management via a WebApp on Dataiku that we implemented. This allowed us to include crucial business know-how provided by those users via a dashboard and used for the optimal CRM allocation of sales leads.
Business Area Enhanced: Marketing/Sales/Customer Relationship Management
Use Case Stage: In Production
Value Generated:
To justify investments into data & analytics the assessment and ongoing measurement of the business impact tracking is absolutely key. We implemented several tools to track the impact and the usage of our analytics solutions, e.g., revenues, product sales as well as adoption and acceptance rates of our solutions by clients and sales agents. The feedback on our analytics solutions we obtain from the sales agents is overall very positive and our insights provide real impact and valuable information to the front.
The number of actionable sales leads we deliver is in the tens of thousands and continuously increases over time, while at the same time we increased our production cycle frequency from semi-annually to now delivering weekly and even daily. We could also extend our scope from covering one business area to now three business areas.
We can attribute a large amount of generated new sales to our machine learning solutions, and we could achieve a significant revenue growth in sales, doubling our impact from the previous year while maintaining a stable team size. The positive ROI of our solution has been recognized by C-level executives and became a key strategic priority.
Value Brought by Dataiku:
Scale:
Dataiku allowed us to scale and to successfully handle our end-to-end production process for generating personalized client insights for 25+ banking products and services and improving millions of client touchpoints across off and online channels. We thereby support thousands of product sales with machine learning insights. We could achieve this while still maintaining a stable team size.
Transparency:
Our team could also profit from the interactive and transparent workbench. The visibility of the flows allows the whole team to have a good overview of different projects in production. The workbench enabled more effective risk management capabilities to ensure smoother production processes. Further, the WebApp functionality allowed us to collaborate more closely and interactively with business users, which further enhances transparency and explainability.
Efficiency:
A huge benefit for our team is the flexibility Dataiku provides. Working mainly with coding recipes and self-written packages, we could easily migrate our previous framework to DSS. We strongly benefit from the scenario scheduling functionality, which increases efficiency of our production cycles and supports us in increasing the frequency of deliveries. Furthermore, visual recipes allow us to quickly test and iterate when developing new models and pipelines. Finally, Dataiku contributed to improving the efficiency of our data pipeline by allowing to ETL from a variety of data sources in a straightforward way.
Upskill:
In general, the DSS platform helped the team to handle model and data maintenance work more efficiently, thus allowing the team to increase the focus on analytics and business insights. The team could also upskill by making use of and learning about the big data tools available on Dataiku, which can be applied in an intuitive and straightforward fashion.