Insights From the Dataiku X ALMA Observatory Volunteer Data Challenge
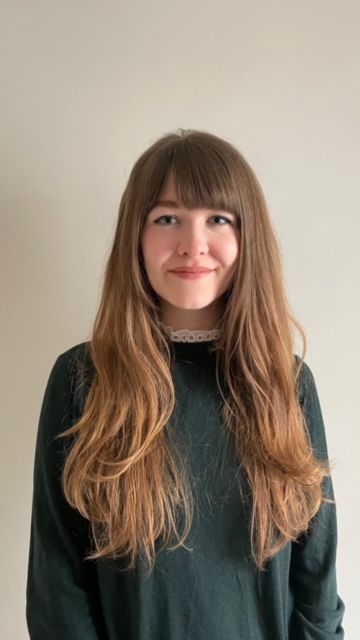
The Atacama Large Millimeter/submillimeter Array (ALMA) is the largest astronomical project in existence, and with a telescope composed of 66 high precision antennas, it produces enormous amounts of data! Since 2017, it’s been Dataiku’s honor to collaborate with the observatory to help its astronomers process this information, thereby optimizing their operations, improving the quality of their observations, and assisting them in their studies of the oldest and coldest objects in the universe.
ALMA’s innovative efforts don’t end there: Their transformation into a data-centric organization made them pioneers in their field, as recognized in the first edition of the Frontrunner Awards.
So when we came up with the idea to create a collaborative hands-on project that would allow analysts from different backgrounds to grow their data science skills together, we couldn’t think of a better partner than ALMA. The end result was an exciting challenge that brought together data science practitioners from around the world, who came together to look at the stars and contribute to a good cause.
Continue reading for a retrospective of the challenge, key results, and insights from our online event which brought participants back together to discuss what they learned!
Overview of the Dataiku X ALMA Challenge
A Celestial Use Case
To provide a foundation for the project, we tasked ALMA with providing us with a data science-related stumbling block they were experiencing, so that the insights generated by volunteers could provide a real impact on the observatory’s work. In response, they delivered the following fascinating use case.
After ALMA’s antennas make an observation, a compute-intensive and long data reduction process starts to produce the final image. That said, the instruments used to perform the observation are not free from sporadic problems and bugs. On top of this, elements such as weather conditions can also negatively affect the data acquisition process.
Example of metadata available per observation (OBSERVE_TARGET being the timing of the observation)
What happens if these problems are not detected before the data reduction starts? A significant amount of processing resources are wasted, and the observation might not be repeated if the object is no longer in the same position in the sky or the required conditions are never met again.
To minimize the probability of this occurrence, ALMA performs a first quality check named Quality Assurance Level 0 (QA0) before the data is processed. This check leverages huge amounts of metadata, which can be analyzed just a few minutes after the observation is done. Most of the process is currently handled manually by an astronomer, who analyzes the metadata with certain criteria to determine its QA0 status: Pass, Semipass, or Fail.
ALMA’s challenge for us? Leverage data science to automatize the QA0 decision, so that it can be made in the least amount of time possible and make more resources available to produce useful observations.
The Challenge in Practice
Our first step to helping find innovative solutions to ALMA’s dilemma was to invite super users, the Dataiku Neurons, and those who had completed certifications on the Dataiku Academy, to partake in our first-ever volunteer project. The goal: To bring together a diverse set of profiles whose unique viewpoints and fresh perspectives could benefit both the observatory and their fellow participants.
In the end, fourteen users from across the globe accepted the challenge. In addition to being equipped with Dataiku, which allowed participants to experiment and build on each other’s work, both Dataiku data scientists and ALMA Observatory staff members were available throughout the process to lend a helping hand.
“I was impressed with the participant's fluency with Dataiku, but it was also fun observing their diligence, creativity, and genuine curiosity around the use case. All of the participants had a ‘full-time life’ outside of this challenge and I was truly inspired by their effort,” says @DarienM
, a Dataiku Data Scientist who provided technical support to our volunteers.
While we initially created the challenge with a structured timeline, it naturally evolved to fit the schedules and interests of participants. A learning experience for all involved – including us – the time and effort put in by the volunteers was also of great benefit to the observatory.
“This project provided ALMA with a fresh perspective to improve on a key component of astronomical observations. We’re very thankful for the work accomplished by volunteers — not only are the insights useful for our day-to-day operations, but they also provide new ideas for future developments in the quality assessment process, and showed us the potential of applying ML and more advanced analytical tools to detect possible failures in advance,” says @Ignacio_Toledo
, Data Analyst at ALMA and Dataiku Neuron, who initiated the project and guided participants throughout.
Results of the Volunteer Challenge
Leveraging Dataiku
To encourage collaboration, we provided a common Dataiku instance for participants to collaborate and learn from each other’s experimentation.
One main project served as the central knowledge hub for the challenge, with the main datasets prepared by Ignacio, as well as a wiki with insights into the methods and processes already in place at ALMA, a basic glossary of technical terms, and summaries of weekly meetings.
Main project… with lots of data!
On top of this, each participant created their own personal projects so they could experiment in a sandbox environment, connecting to the datasets as needed to complete their tasks with their preferred technologies and techniques.
“Inside your own project, you can do some exploratory analysis, write some custom Python code, or easily join, filter, or aggregate data,” says Pauline van Nies (@pvannies
), Data Scientist at Ordina NL. “You can use the functionalities of Dataiku to make charts directly from the datasets, or choose to write in your own notebook, which has the advantage of allowing you to write your ideas down, so you can easily share them later on during a discussion or via a link on Slack.”
Jupyter notebook created by Ignacio to help participants query and parse data from antennas in order to identify anomalies
Outlier Detection
To start off the challenge, participants were first tasked with exploratory data analysis, with the goal of identifying outliers – that is, determining what results in a “bad observation” in order to flag it in the system.
Pauline found that, unlike previous assumptions, not all antennas were behaving in the same way. This key finding prompted her to conduct further data analysis on single antennas rather than on the assembly. She explains:
“Combining ideas from Jordan [Blair, Solutions Engineer at Schlumberger] and Giuseppe [Naldi, Software Architect at ICTeam], I performed an outlier detection and a changepoint algorithm using Python on a dataset of 17.5 million records. To visualize the results of the analysis, I created a Dash/Plotly webapp that can support the engineers of ALMA to investigate the historical antenna behavior and its changes, which can be applied in a predictive maintenance use case.”
Chart showing the automatic detection of variations in the temperature of a component in one antenna, using the change point algorithm
To further anomaly detection, Giuseppe (@gnaldi62
) used statistical methods and introduced participants to different machine learning algorithms, with outstanding results: “I think that my main contribution to the project has been to suggest the application of a changepoint detection algorithm to the analysis of the time series. I had found such analysis useful in other contexts (e.g., anomaly detection or predictive maintenance) so I did believe it could have been useful also in this specific context.”
Chart showing anomaly detection using an isolation forest algorithm
Webapp Visualization
Niklas @Muennighoff
, Data Scientist at Dataiku, volunteered his time to our challenge to build a visual representation of the insights discovered by participants. He accomplished this through the creation of a web application (webapp), which enables the astronomer on duty to analyze an individual observation for quality assurance.
Webapp demonstration
Niklas further elaborates: “I built a webapp for visualizing and interacting with observation data from ALMA. The webapp allows users to look at an observation in aggregate or drill down to the level of single antennas, basebands, temperatures, frequencies & other parameters. It makes use of a combination of SQL, Python (Pandas, Dash), and CSS. Thanks to the work of Ignacio [Toledo], the webapp has been deployed at ALMA. Via Dataiku Scenarios, it automatically pulls the latest observation data so astronomers can perform the QA0 check within three minutes of the observation.”
Key Learnings & Final Thoughts
As we hope to launch other volunteer challenges in the future, collecting feedback from the participants of our first endeavor was vital. In addition to anonymous surveys, we held a final meeting before the project officially wrapped up to allow participants to reflect and share their main takeaways, with multiple contributors highlighting the opportunity to learn from each other, volunteer for a good cause, and use interesting data sets from ALMA. To our delight, there was also unanimous interest in participating in the next challenge, for which we have taken note!
“It's not every day we have the opportunity to work with astronomers on such a project. ALMA is unique in the world, and I feel very lucky to have been part of it,” says Matthieu Scordia (@Mattsco
), Lead Data Scientist at Dataiku.
Tom Brown (@tgb417
), a data scientist and Dataiku Neuron who has both participated in and organized volunteer challenges, was in agreement: “I enjoyed participating. In a strange way this was a humbling experience for me. I’m usually a solo practitioner in an industry that is not super sophisticated in the use of its data. So I’m often a leader on the project I’m doing. In this case I got to interact with folks who are really doing data science. In an area of personal passion, astrophysics and space science.”
Screenshot from our Dataiku X ALMA Challenge online event, where participants came together to share what they learned with the community
While the challenge was a success, it was still the first large scale volunteer project that we had organized, and as such we underwent a learning curve. Feeling we had knowledge to share, we decided to make this the focal point of a follow-up online event, which brought our participants back together to reflect on the project and discuss the value and challenges of volunteer data science collaboration.
Watch a recording of the event below to hear directly from our organizers and volunteers!
//play.vidyard.com/sKnq4xn4q4kwWp6LVP4hwx.html?
Would you participate in our next volunteer challenge? If so, why? Let us know in the comments – we’ll take your feedback into consideration when planning our next project!