NEC Networks & System Integration Corporation - Explainable Forecasting Figures to Inform Sales and
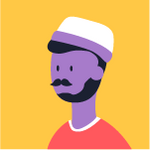
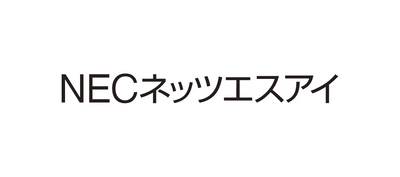
Team members:
- Takuya Minegishi
- Ryotaro Suzuki
- Ginji Ichikawa
- Junki Toyokawa
- Yushi Minami
Country: Japan
Organization: NEC Networks & System Integration Corporation
NEC Networks & System Integration Corporation provides a wide range of services, from ICT and networking to installation and maintenance, to a variety of customers, including corporations, telecommunications carriers, government agencies, and social infrastructure providers.
Awards Categories:
- Best Acceleration Use Case
- Best Data Democratization Program
- Best ROI Story
Business Challenge:
Our main business is designing and installing PBX and networks for both private and public customers in Japan. In recent years, we have been also focusing on reselling SaaS in addition to offering system integration and O&M services.
One symbolic example would be we are the first Zoom distributor in Japan. Due to the global pandemic, demand for SaaS resales, SI, and operations, including Zoom, temporarily surged in Japan. However, as the pandemic subsided, the demand for these services began to slow down.
Under such circumstances, it is now imperative for us to forecast demand in each business area, reexamine human resource allocation, and logically formulate GAP measures to meet budget targets. In addition, the amount of time spent on forecasting and simulation is extremely large, making it necessary to improve operational efficiency.
Until now, the person in charge of the planning department of each business division in our company has forecasted the final order amount using Excel and other tools based on experience and intuition, using current prospects and past trends, and if the budget is likely to fall below the target value, he or she will consider measures while being pressed by daily budget progress management work.
However, there were several issues in this process, including the following.
- Poor explanatory power of forecast figures
- Calculation of forecast figures is too personalized.
- The frequency of forecasting is not timely.
- Lack of concrete action strategies to acquire new projects.
- Inability to grasp the risks of priority projects and implement countermeasures in a timely manner.
Business Solution:
We chose Dataiku because it allows our team to deliver results quickly and with less stress. Dataiku enables data preprocessing, feature engineering, machine learning, and operational monitoring of models in a single application. All data preprocessing was handled by Dataiku recipes, while Python connectors were used for the output method and some modeling of the predictive results of the learning models.
The project involved working with one data scientist, three data engineers, and members of the end-user sales and business departments to solve the problem by building the following models, but the first modeling was created in just one month.
Nearly 100 recipes and 8 models were built, and by using automation nodes, the data is updated daily, the models are updated, and operations are monitored.
1. Demand forecasting model
By providing future demand forecasts based on past performance, we assist end-user sales and business divisions in mid- to long-term strategic planning, human resource allocation, and business strategy consideration.
2. Action suggestions using the prospect's order probability model and inverse analysis
It extracts 500,000 past prospect data and detailed sales activity data from SFA/CRM and automatically determines the probability of receiving an order at an ongoing prospect, thereby supporting short-term strategy review. Specifically, we provide suggestions for prospects and next actions to focus on.
We will consult with end-users and conduct feature-volume engineering over and over again, The suggestions are also presented in the form of "action advice from the AI. The suggestions are presented in the form of "action advice from AI," and are worded in a way that makes it easy for the user to take action. This system is more user-friendly.
3. Positive-negative determination of a prospect
Analyzes sales representatives' reports using natural language processing, By using natural language processing to analyze the sales representative's report and automatically determine whether it is positive or negative, Alerts managers and executives quickly of potential risks for focused prospects. The system was built using Dataiku's plug-in functionality, which made it quick and easy to deploy.
Day-to-day Change:
These models provide explainable forecasting figures, which are updated daily and available for quick action by the sales and business divisions. Benefits include:
- Quickly identify prospects to focus on and prioritize based on projected order probability.
- Identify specific action strategies to win contracts.
- Factors contributing to the order rate can be interpreted.
- Order accuracy and positive negatives can be used to diagnose prospecting from multiple angles.
- Significant reduction in man-hours required for forecasting and numerical simulation, which used to be done manually.
Business Area Enhanced: Marketing/Sales/Customer Relationship Management
Use Case Stage: In Production
Value Generated:
The business division planning department, one of the end users, is responsible for analyzing and forecasting the business division's performance, and then formulating measures and strategies to improve sales and profitability, However, the analysis and calculation of forecast figures requires an enormous amount of time (20,000 hours per year).
The model constructed by Dataiku not only significantly reduces the time required, but also improves the accuracy of the forecasting process, The model developed by Dataiku not only saves a great deal of time, but also boasts higher accuracy in forecasting, In addition, you will be able to spend more time on strategic planning, which should be your main focus.
Value Brought by Dataiku:
1. Rapid data processing and model building
Visual recipes are an excellent feature, and without advanced data science or programming knowledge, machine learning models can be built quickly without advanced data science or programming knowledge.
2. End-to-end automation
It can automatically build models with multiple algorithms, compare them, automatically select the best model, and automatically manage model versions, Automatic model versioning, visual and flexible threshold settings, and more.
There are too many attractive features to enumerate in each process of data preparation, model building, and evaluation.They have significantly shortened the development man-hours during the project execution.
3. Plug-ins, Business Solutions
By using various plug-ins and business solutions The use of various plug-ins and business solutions allows for the rapid output of higher value-added analyses and outputs.
Value Type:
- Increase revenue
- Reduce cost
- Save time
Value Range: Hundreds of thousands of $