ALMA Observatory (on behalf of volunteers) - Fostering Collaboration From All Backgrounds For a Good
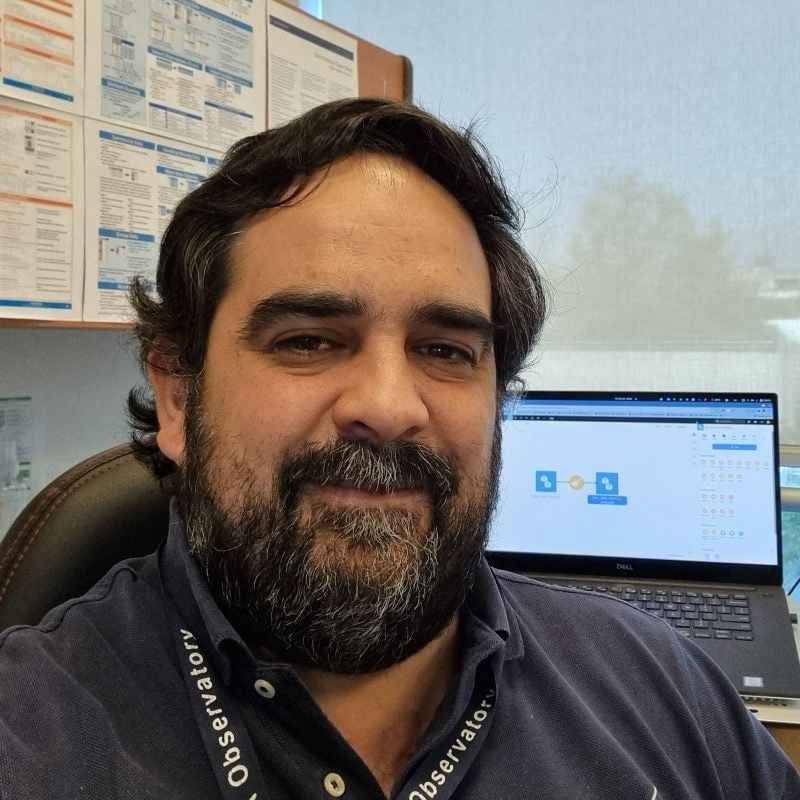
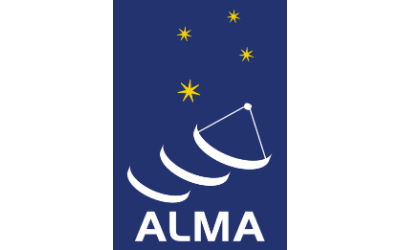
Names:
Ignacio Toledo, Data Analyst, Data Science Initiative Lead, ALMA Observatory
Pauline van Nies, Data Scientist, Ordina NL
Giuseppe Naldi, Software Architect, ICTeam
Niklas Muennighoff , Software Engineer, Dataiku
Tom Brown, Director of Data & Analytics, 41xRT
Jack Craft, Independent Consultant
Jordan Blair, Solutions Engineer, Schlumberger
Akshay Katre, Data Scientist, Rabobank
Marc Robert van Luijpen, Data Scientist, Ordina NL
Bruno Carvalho, Porsche Consulting
Josselin Tobelem, Data Scientist, LeBonCoin
Manikandan Sree, Consultant, Tech Mahindra
Mukunth Rajendran, Johnson & Johnson
Celia Verdugo, Science Operations
Kurt Plarre, Science Operations, ALMA Observatory
Matias Radiszcz, Science Operations, ALMA Observatory
Rosita Hormann, Software Engineer, ALMA Observatory
Jorge García, Science Archive Content Manager, ALMA Observatory
Takeshi Okuda, Senior Instrument Engineer, ALMA Observatory
Matthieu Scordia, Lead Data Scientist APAC, Dataiku
Darien Mitchell-Tontar , Data Scientist, Dataiku
Lisa Bardet, Head of Advocacy, Dataiku
Country: Chile
Organization: ALMA Observatory
The Atacama Large Millimeter/submillimeter Array (ALMA) is an international partnership of the European Southern Observatory (ESO), the U.S. National Science Foundation (NSF) and the National Institutes of Natural Sciences (NINS) of Japan, together with NRC (Canada), NSC and ASIAA (Taiwan), and KASI (Republic of Korea), in cooperation with the Republic of Chile. ALMA -the largest astronomical project in existence- is a single telescope of revolutionary design, composed of 66 high precision antennas located on the Chajnantor plateau, 5000 meters altitude in northern Chile.
Awards Categories:
- Data Science for Good
- Moonshot Pioneer(s)
- Most Impactful Ikigai Story
- Most Extraordinary AI Maker(s)
Business Challenge:
The Atacama Large Millimeter/submillimeter Array (ALMA) is the largest earth-based astronomical observatory. With a telescope composed of 66 high-precision antennas located on a high-altitude plateau in Chile, we produce terabytes (and soon petabytes) of data every year.
We have been collaborating with Dataiku since 2018 to become one of the first observatories to leverage data - not only for scientific research, but also to optimize our operations, so as to spend more of our limited time and resources to improve the quality of our observations.
The Dataiku Ikig.ai program provides the platform and ad hoc resources to drive this organizational transformation. With the Dataiku Community, we also had the opportunity to entice volunteer users to contribute to our mission.
A major data-related challenge that we currently encounter relates to quality checks of the observations. After ALMA’s antennas perform an observation, a compute-intensive and long data reduction process starts to produce the final image. But the instruments used to perform the observation are not free from sporadic problems and bugs, and elements such as weather conditions can also negatively affect the data acquisition process.
If these problems are not detected early on, a significant amount of processing resources are wasted to vainly attempt at making the observation ready for scientific research. This process is called “data reduction”, as it pertains to forming the best-quality possible image based on multiple “layers” of observation, including light spectrum.
In addition, it may cause a missed opportunity - as the observation might not be repeated if the object is no longer in the same position in the sky or the required conditions are never met again.
To minimize the probability of this occurrence, ALMA performs a first quality check named Quality Assurance Level 0 (QA0) before the data is processed. This check leverages huge amounts of metadata, which can be analyzed just a few minutes after the observation is done. Most of the process is currently handled manually by an astronomer, who analyzes the metadata with certain criteria to determine its QA0 status: Pass, Semipass, or Fail.
We set out to research whether data science could be leveraged to automatize the QA0 decision so that it can be made in the least amount of time possible and make more resources available to produce useful observations.
Business Solution:
The Dataiku Community team invited super users from the Dataiku Neurons program, as well as those who had completed certifications on the Dataiku Academy, to partake in our first-ever volunteer project.
14 users from across the globe accepted the challenge. They were provided with a common Dataiku instance to collaborate and learn from each other’s experimentation, and supported by both Dataiku data scientists and ALMA Observatory staff members.
As a first step toward QA0 automation, and after some iterations between the volunteers and the ALMA experts, we decided to identify what makes a qualitative vs. useless observation through outlier detection
1. Outlier Detection
Participants were tasked with exploratory data analysis, with the goal of identifying outliers among the different variables influencing an observation.
@pvannies
, Data Scientist at Ordina NL, found that not all antennas were behaving in the same way, unlike previous assumptions. This key finding prompted her to conduct further data analysis on single antennas rather than on the assembly. Combining ideas from Jordan Blair, Solutions Engineer at Schlumberger, and Giuseppe Naldi, Software Architect at ICTeam, she performed an outlier detection and a changepoint algorithm using Python on a dataset of 17.5 million records. To visualize the analysis results, she created a Dash/Plotly webapp that can support the engineers of ALMA to investigate the historical antenna behavior and its changes, which can be applied in a predictive maintenance use case.
To further anomaly detection, @gnaldi62 used statistical methods and introduced participants to new ML algorithms, such as isolation forest and changepoint detection, to perform a time series analysis.
Chart showing anomaly detection using an isolation forest algorith
2. Webapp Visualization
Niklas @Muennighoff, Data Scientist at Dataiku, volunteered his time to our challenge to build a webapp featuring the insights discovered by participants. The astronomer on duty may use it to analyze an individual observation for quality assurance, either in aggregate or at the level of single antennas, basebands, temperatures, frequencies, and other parameters.
The webapp was made using the Dash framework with Plotly visualization components, and CSS was used to create a custom design to make the tool more appealing to ALMA users.
Thanks to Dataiku capabilities, the webapp frontend is integrated into a "Dataiku project", and can query, using SQL, all the data already prepared by a flow, which is automatically refreshed using a scenario that is triggered when new observations are available. The outcome is that the deployment of the webapp is quick, and it doesn't require overly complex DevOps processes to be put in production.
Value Generated:
The webapp was deployed at ALMA and is now a tool available at the fingertips of the astronomer on duty to check the main quality indicators of the latest observations produced. Within three minutes of the observation, the data is visible within the webapp and enables the astronomer to quickly notice any anomalies. The interactive experience is improved in regard to the traditional platform used, and provides more perspectives around the data.
While we initially set out to potentially automatize the entire quality check process, we learned along the way that there are many different indicators that make (or break) an observation. Around 20 different tables and 50 different variables must be checked - and some of those variables are checked antenna by antenna, meaning that hundreds of metrics must be analyzed.
Furthermore, this was the first time that the data from different observations could be analyzed together, in order to find trends and outliers that not only can provide us information about a failure of a particular observation, but also can help us forecast problems with some of the antennas or their components, allowing us to take corrective actions in advance.
Our next challenge should hence first focus on determining which parameters have the biggest positive and negative influences on the quality of the observation.
All 14 participants, as well as ALMA staff and Dataiku volunteers, have learned tremendously along the way on a number of different aspects:
- The nitty-gritty of astronomical research: starting with understanding the technical jargon (e.g. basebands, polarizations) to the logistics involved with piloting 66 high-tech antennas (and many of the components which need to work perfectly together), as well as the influence of external factors, and finally the human organization behind leveraging all of this for powering the next big scientific discoveries. Thank you to all ALMA staff volunteers who have shared their knowledge and insights along the way!
- Analytics and machine learning, through sharing ideas and progress in our weekly calls. It was incredibly inspiring to brainstorm the most efficient way to clean 17.5 million records, discuss the pros and cons of different possible algorithms for outlier detection, and finally agree on how to present the insight in a way that is most useful to astronomers. Thank you to the participants for taking the time to contribute their skills, and laughs!
- Lastly, collaborating was the most favorite part of the project for all involved. We’ve made a big impact in high spirits with 14 strangers from around the world, all united through common goals of helping ALMA and learning from each other. We set up our own mix of synchronous and asynchronous communication, divided tasks depending on interests, and made it work all together!
Business Area Enhanced: Product & Service Development
Use Case Stage: Built & Functional
Value Brought by Dataiku:
Dataiku was pivotal to enable volunteers and observatory staff, from different skills and backgrounds, to collaborate on a single visual, accessible interface.
We’ve created one main project, which served as the central knowledge hub for the challenge. It contained the main datasets prepared for analysis by @Ignacio_Toledo
(Data Analyst at ALMA), as well as a wiki with insights into the methods and processes already in place at ALMA, and a basic glossary of technical terms as well as summaries from our weekly meetings.
On top of this, each participant created their own personal projects, so they could experiment in a sandbox environment, connecting to the datasets as needed to complete their tasks with their preferred technologies and techniques. All these projects were available for other participants to peek in, so they could learn from each other and help out when asked. We had a complementary Slack channel for more immediate discussions and Q&As.
It was easy to add new datasets along the way, we’ve quickly added new packages and environments as participants asked for new technologies, such as Dash. Resources like the Dataiku Academy also enabled them to upskill quickly on their areas of interest, for instance to contribute to the webapp.
The project was born out of the strong partnership between ALMA and Dataiku, and we look forward to many more of these exciting and helpful projects!
Value type:
- Improve customer/employee satisfaction
- Reduce risk
- Save time