Controlling Data Quality: Tips and Tools
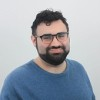
Originally posted by Dataiku on April 19, 2021
Only 8% of CDOs are content with the quality of data at their disposal. Data needs to be valuable, thus of high quality, to drive machine learning model success.
In a recent Egg On Air Episode, Jeff McMillan, Chief Analytics and Data Officer for Morgan Stanley Wealth Management, outlined the significance of data quality to an organization’s success and offered some insight on how Morgan Stanley approaches data quality.
Considerations for Controlling Data Quality
Jeff McMillan cites data quality as one of the decisive factors to becoming an intelligent organization. Let’s start by listing a few things you need to have in place to control your data quality:
- Data quality infrastructure
- Metrics around accuracy
- A clear definition of what “quality” means to your organization
- People who are accountable for the accuracy and in charge of monitoring data quality on a daily basis Issues management control
"A lack of quality data is probably the single biggest reason that organizations fail in their data efforts."
While there are some smart, automated ways to help improve data quality, it's not a magic bullet.
An Organizational Problem
Most organizations do not have accurate product, pricing, or client information. And even when the information is accurate, it is often not consistent or simply not accessible in any simple way. The problem of data quality is not always a technological one — sometimes it’s an organizational one.
Teams need to decide who will be in charge of what and assign the role of setting clear definitions, metrics, categorization rules, and goals to specific individuals. For example, who will be evaluating data quality and will this evaluation be based on completeness, validity, timeliness, etc.? The first step to reach accuracy and consistency is to clearly define these roles and responsibilities. The next step revolves around putting in place additional data democratization and collaboration efforts, starting with data centralization.
Data Centralization
A centralized data repository is almost essential to being successful with your data quality strategy. A central location not only helps distributed or remote teams work more efficiently by providing one clear data resource point, thus increasing accessibility, but it also helps manage consistency and accuracy.
Having multiple sources of truth may lead to finding different values for one same statistic or other inconsistencies, so organizations have to determine which attribute they believe to be the single source for a customer record, product record, etc. Only then can you begin to discuss accuracy, consistency, timeliness, and other concerns.
"If you don't have accurate data, nothing else works."
While there are some smart, automated ways to help improve data quality, it's not a magic bullet.
How Morgan Stanley Solves the Issue of Data Quality
Morgan Stanley has made phenomenal progress in many of its projects, such as their Next Best Action initiative, the sophisticated algorithms they are using, their work around predictive analytics and data visualization, and more. However, the real driver of this success is the work that has been done around data quality. The organization has put in place:
- Data stewards who are accountable for data accuracy
- Data quality engines that turn every night
- An issues management process
- Data definitions that are put into their systems which everyone can access
Monthly governance meetings - A governance infrastructure that can take in any data quality problem that arises, evaluate it, and determine which action must be taken as well as the appropriate resources to address it
"Data quality is potentially the single most important factor in success."
These strong data quality efforts have taken Morgan Stanley about five years to implement in a meaningful way and today make up one of the company's competitive advantages.
Generate High-Quality Data With Data Labeling
Uncover how high-quality data can lead to high-quality model performance, specifically through the lens of data labeling and active learning.