Importance of Data Engineering Use Cases in Documentation
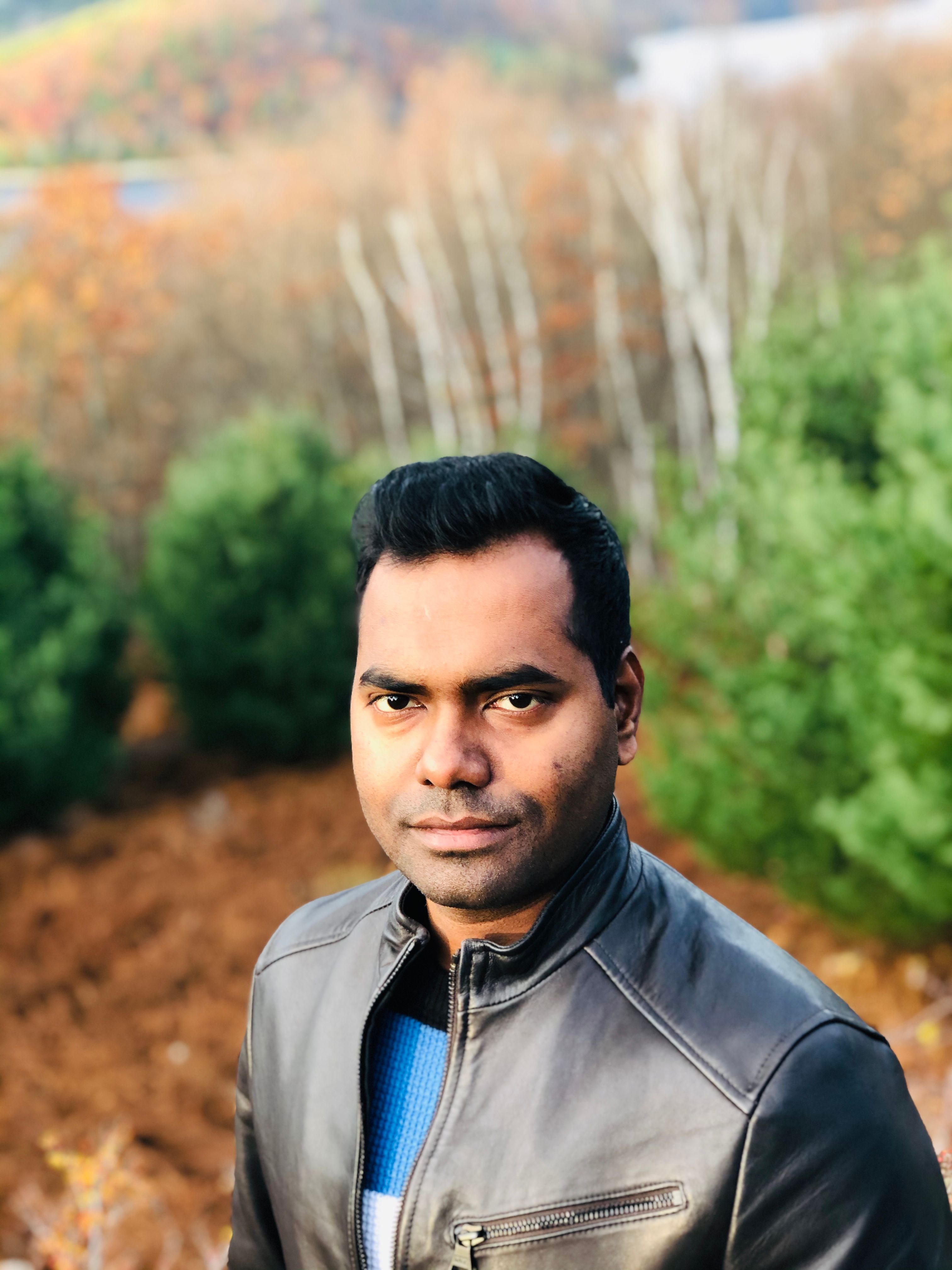
Fortunately, I have had experience in working with huge number of CSV files -- Big data solution. I have noticed customers are more keen to perform data prep in other platforms and consider Dataiku for insights, analytics, data science and MLOps. Dataiku has potential to leverage data engineering aspect as well. I imagine how it could be convenient if everything comes under single umbrella -- Dataiku!. In order to achieve that, I believe that Dataiku needs to publish documents on data engineering activities and best practices of underlying infrastructure, which I think is most important.
If customer can relate with use cases that Dataiku already successfully performed and provided benefits to various sectors, it will obviously have better confidence in adopting Dataiku.
Comments
-
It’s not rare that a data engineer is confused with a data scientist. We asked Alexander Konduforov, a data scientist at AltexSoft, with over ten years of experience, to comment on the difference between these two roles: “Both data scientists and data engineers work with data but solve quite different tasks, have different skills, and use different tools. Data engineers build and maintain massive data storage and apply engineering skills: programming languages, top budget home theatre speakers ETL techniques, knowledge of different data warehouses and database languages. Whereas data scientists clean and analyze this data, get valuable insights from it, implement models for forecasting and predictive analytics, and mostly apply their math and algorithmic skills, machine learning algorithms and tools.” Alexander stresses that accessing data can be a difficult task for data scientists for several reasons. Vast data volumes require additional effort and specific engineering solutions to access and process them in a reasonable amount of time. Data is usually stored in lots of different storages and formats. In this case, it makes sense first to clean it up by taking dataset preparation measures, transforming, merging, and moving to more structured storage, like a data warehouse. This is typically a task for data architects and engineers. Data storages have different APIs for accessing them. In this case, data scientists need data engineers to implement the most efficient and reliable data pipeline for their purpose. As we can see, working with data storage built by data engineers, data scientists become their “internal clients.” That’s where their collaboration takes place.