MandM - Managing Models at Scale to Deliver Faster Insights
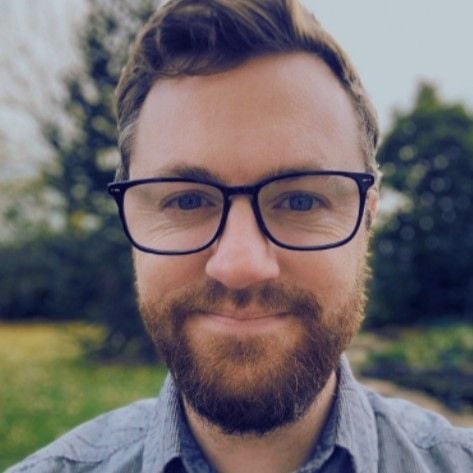
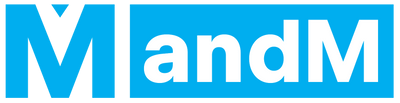
Team members:
Ben Powis - Head of Data Science
Joel Lenden - Junior Data Scientist
Tobi Osinowo - Data Scientist
Jim Taylor - Data Analyst
Oisin Devitt - Data Analyst
Country:
United Kingdom
Organization:
MandM
Description:
Our journey began in 1987 by founders Mark Ellis and Martin Churchward (the 'two Ms'), selling end of line sports products directly to customers in the UK.
Now more than 30 years on, we're now one of Europe's leading online off price retailers - with over 2 million active customers. We have dedicated local market websites in Ireland, Germany, France, Netherlands, Denmark and Poland as well as dispatching to another 20+ countries worldwide.
Our success is down to our commitment and passion for seeking out the biggest fashion, sport and outdoor brands at unbeatably low prices all year round, to make sure you get even more for your money.
Awards Categories:
- AI Democratization & Inclusivity
- Value at Scale
Challenge:
MandM is one of the largest online retailers in the United Kingdom with over 3.5 million active customers and seven dedicated local market websites across Europe. The company delivers more than 300 brands annually to 25+ countries worldwide - which means that in 2020, we grew fast. This meant more customers and, therefore more data, which magnified some of our challenges:
- Getting all the available data out of silos and into a unified, analytics-ready environment:
The core data team is made up of four people (two data scientists, and two data analysts), but we extend our reach by leveraging a hub and spoke model for our data center of excellence, meaning we work with analysts embedded across the business lines to scale our efforts. However, this requires an easy way to enable those teams to leverage data to answer business questions that doesn’t necessarily involve code.
- Scaling out AI deployment in a traceable, transparent, and collaborative manner:
MandM’s first machine learning models were written in Python (.py files) and run on the data scientist’s local machine, and we needed a way to prevent interruptions or failure of the machine learning deployments.
In an attempt to tackle the second challenge, our team moved these .py files to Google Cloud Platform (GCP), and the outcome was well received by the business and technical teams in the organization. However, once the number of models in production went from one to three and more, we quickly realized the burden involved in maintaining models. There were too many disconnected datasets and Python files running on the virtual machine, and we had no way to check or stop the machine learning pipeline.
Solution:
We turned to the powerful combination of Dataiku and GCP to answer these critical challenges. With Google BigQuery’s fully-managed, serverless data warehouse, we were able to break the data silos and democratize data access across teams. MandM was one of the first online retailers to implement Google BigQuery across the organization.
At the same time, thanks to Dataiku’s visual and collaborative interface for data pipelining, data preparation, model training, and MLOps, our team could also easily scale out the models in production without failure or interruptions - all this in a transparent and traceable way.
MandM now has hundreds of live models doing everything from scoring customer propensity to generating pricing models, all with visibility into model performance metrics, clear separation of design and production environments, and many more MLOps capabilities built into the platform.
Teams can now easily push-down and offload computations for both data preparation and machine learning to GCP. Using Dataiku means this capability is accessible to all user profiles across the organization, without knowing the underlying technologies or complexity.
We love the flexibility offered by Dataiku. We do have a mix of people that go more toward AutoML and visual tools as well as one data scientist who loves to work in code. That’s the beauty of the platform and why we chose it — we didn’t want a low-code tool where we could get lazy and just click a few buttons. Now the team has the best of both worlds: if they want to nerd out and go under the hood, they can do that. If they need a quick model, they can do that too.
Impact:
The benefits we have seen by using Dataiku and GCP aren’t limited to time saved from tedious maintenance work - we’re also having more impact across the business. Since we began our journey with Dataiku in January 2020, 54 projects were created, which handle 1,171 different datasets and are orchestrated by 53 different scenarios, making sure our models build only when the data is available and validated. We have 9 large projects deployed to an automation node, which are solving complex business problems or providing advanced insight on a daily basis.
Our data team is now able to deliver a variety of solutions on business problems from adtech to customer lifetime value, whether that’s a dashboard, a more detailed piece of analysis or a machine learning project deployed in production.
For example, one application might be business users in the buying and merchandising teams, who could interact with machine learning models in their day-to-day work through Dataiku applications, which provide a nontechnical interface for projects developed by the data team.
We’ve also built out a feature library with Dataiku that contains more than 400 features specific to MandM’s business. Now, the feature library is the first place people go, sort of like a shop window for machine learning projects — it takes away the monotony and repetition of their work.
Having a platform like Dataiku allows our data scientists to focus on building cool things, not spending hours and hours on maintenance and making sure things are running. With workflows deployed in Dataiku, we save days of work every month.