HES-SO - Teaching the Next Generation of Chief Data Officers with Dataiku
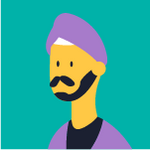
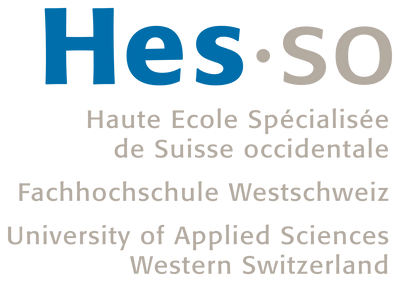
Team members:
Cédric Gaspoz, Professor UAS
Dominique Genoud, Professor UAS
Country:
Switzerland
Organization:
University of Applied Sciences and Arts Western Switzerland (HES-SO)
Description:
HES-SO is a network of 28 schools of higher education offering degree programmes in six key fields to some 21,000 students. Our universities play a key role in the social, economic and cultural development of each of western Switzerland’s seven cantons. The Master of Science in Business Administration (MSc BA) gives the opportunity to develop the understanding of management they acquired during their Bachelor’s course and specialise in a fast-growing area of competence.
Awards Categories:
- Excellence in Teaching
Challenge:
To accompany our students and their future employers in the digital transition, we have thoroughly revised our business intelligence courses. Our students must not only be able to analyze data, but also to become information producers with all the steps that this includes.
During the three BI courses of the master, we start by refreshing the knowledge of R, before starting the discovery of data science that leads us from data acquisition to Deep Learning. It was possible to introduce the students to the different types of training and evaluation of the models by using the available metrics and data splitting that are usually used in machine learning. The built-in graphical explanations about the results greatly facilitated the understanding of the tuning of the models and their understanding.
Another challenge we wanted to address with this redesign was the production stage. Often, curricula stop at the learning of models and their evaluation. However, from a business point of view, it is only when the models are deployed that we start to create value. It was therefore important to be able to concretely see how to use the models to support business processes.
When redesigning these courses we faced several challenges:
- Multiple tools implemented depending on the languages (R or Python)
- Tools dedicated to only one part of the workflow (data cleaning, machine learning...)
- Lack of tools for the release of models in production
- Lack of understanding of the metrics used to check the quality of the models in production
- No possibility of collaboration between students on the same project
- Feedbacks and corrections take a lot of time (file transfer between students and teachers)
- IT support for multiples tools
The lack of integration of the tools also prevented us to successfully proposing integrative pedagogical scenarios because it was difficult to actively collaborate with several people on the same task.
Solution:
During our review of different tools, we had the opportunity to test Dataiku. The ability to support all phases of the lifecycle as well as the integration of notebooks convinced us to pursue the discussions with the Dataiku academic team. The most important weakness was the absence of the API services in the academic offer, which Dataiku finally integrated into its offering.
Our Dataiku instance has been deployed in our global infrastructure on Azure and is perfectly integrated in our processes (incl. provisioning, authentication...).
After one year of classes, we have 109 users in 39 groups who have produced 646 projects, 2,491 recipes, 614 notebooks, 752 models and 67 API services.
This usage includes exercises and work done in class, individual projects, group projects, a hackathon and some master's thesis. Using the Dataiku API allows us to efficiently create projects, assign rights, track progress and evaluate results.
As teachers, especially in the pandemic year, Dataiku allowed us to support all teaching activities. The first discovery of Dataiku was through the R notebooks. By revisiting the statistical basics and the R language, students started to use the dataset features. Then, through a day animated by a data scientist from Datailu, the students discovered the preparation and classification of data with the integration of R recipes. As the weeks went by, we introduced more advanced notions to finish with image recognition using deep learning. Finally we saw how to publish a model using the API services and integrate it using a simple webapp, also created in Dataiku.
Various group projects allowed the students to put their knowledge into practice on different datasets related to concrete business problems (e.g. sales prediction, churn, audit, mortgages). The groups performed all the tasks related to the lifecycle: data preparation, feature creation, feature selection, model learning, hyperparameter selection, selecting the best model, deploying the model on the API services, and querying the model with a webapp:
Impact:
The course content is organized around the tasks of the CDO (Chief Data Officer). In collaboration with CDOs, who are also involved in the course, we have defined 26 user stories that cover all aspects of the function. While the theoretical aspects are covered in a more traditional way, the practical aspects are realized on Dataiku. Thus, without being data scientists, the students had the opportunity to concretely explore the different aspects of the job and to implement them through various use cases. This allows them to specialize in data science or in managerial functions where they will be required to manage the different aspects of data projects within multidisciplinary teams.
Because of the importance of practice during the courses, we have adapted the assessments to allow students to be in a situation close to reality. At the end of the course, a hackathon was organized with the goal of developing a webapp for investors, allowing them to determine the financing opportunities, based on a dataset on the success of startups according to the financing rounds.
During 10 hours, groups of students from management and computer science departments had to complete 15 tasks (data balance analysis, feature creation with R, subpopulation analysis, ...) and produce 10 deliverables (map of failures and geographical disparities of factors, evaluation of model results, ...). A board meeting was also organized in the middle of the day to review the intermediate results and distribute new data.
It should also be noted that the 10 groups (40 students) had to work remotely due to health restrictions, which would not have been possible without a tool such as Dataiku. At the end of the day, the groups were able to present their results and demonstrate their webapp based on the best trained model.
After the first iteration, student satisfaction was very high and the Dataiku tool was quickly adapted. Several students chose to do their master thesis using Dataiku.
Quotes from the course evaluation:
- “Really cool to have discovered and tested R and Dataiku. Thanks for the Hackathon experience and the whole organization!”
- “Very rich content, intervention of a Dataiku data scientist.”
- “Very interesting material and dynamic presentation, good alternation between theory and exercises.”
The knowledge of Dataiku by our students allowed us to propose master thesis subjects including advanced machine learning algorithms. As the Dataiku tools were sufficiently well understood, many students have chosen subjects containing machine learning, ranging from sleep cycle analysis to recognition of objects on geographical maps, and face recognition. They will all use the features provided by the Dataiku framework.Number of distinct users per day - showing a strong interest in Dataiku, even outside of the class!